Vision-Aided 6G Wireless Communications
An overall block diagram illustrating the future blockage prediction problem and our proposed machine learning-based solution. The proposed solution takes in a sequence of bimodal image-beam tuples and produces a prediction of the future blockage status
Key ideas
- A promising way to tackle the reliability and latency challenges in mmWave/THz communication systems lies in enabling proaction in wireless networks. Proaction allows the network to anticipate blockages, especially dynamic blockages, and initiate user hand-off beforehand.
- Additional sensory data such as RGB images captured by the mmWave base station can provide a sense of the surroundings in a wireless environment. The side information provides the network with the necessary information to identify different objects of interest in the environment and their behavior. This, in turn, enables the network to proactively predict future blockages and perform hand-off, thereby increasing the reliability of these systems.
- The paper develops a vision-aided wireless communication framework that utilizes machine learning models and the dual-modality of visual and wireless data to perform proactive blockage prediction and user hand-off.
Applications
- Enabling future mmWave/THz communication systems to predict future blockages and manage hand-off/beam-switching decisions proactively
- Reduce initial access latency, increase reliability and resource utilization efficiency of future mmWave/THz networks
More information about this research direction
Paper: Gouranga Charan, Muhammad Alrabeiah and Ahmed Alkhateeb, “Vision-Aided 6G Wireless Communications: Blockage Prediction and Proactive Handoff,” in IEEE Transactions on Vehicular Technology, vol. 70, no. 10, pp. 10193-10208, Oct. 2021.
Abstract: The sensitivity to blockages is a key challenge for millimeter wave and terahertz networks in 5G and beyond. Since these networks mainly rely on line-of-sight (LOS) links, sudden link blockages highly threaten the reliability of the networks. Further, when the LOS link is blocked, the network typically needs to hand off the user to another LOS basestation, which may incur critical time latency, especially if a search over a large codebook of narrow beams is needed. A promising way to tackle the reliability and latency challenges lies in enabling proaction in wireless networks. Proaction allows the network to anticipate future blockages, especially dynamic blockages, and initiate user hand-off beforehand. This article presents a complete machine learning framework for enabling proaction in wireless networks relying on visual data captured, for example, by red-green-blue (RGB) cameras deployed at the base stations. In particular, the article proposes a vision-aided wireless communication solution that utilizes bimodal machine learning to perform proactive blockage prediction and user hand-off. This is mainly achieved via a deep learning algorithm that learns from visual and wireless data how to predict incoming blockages. The predictions of this algorithm are used by the wireless network to proactively initiate hand-off decisions and avoid any unnecessary latency. The algorithm is developed on a vision-wireless dataset generated using the ViWi data-generation framework. Experimental results on two basestations with different cameras indicate that the algorithm is capable of accurately detecting incoming blockages more than ~90% of the time. Such blockage prediction ability is directly reflected in the accuracy of proactive hand-off, which also approaches 87%. This highlights a promising direction for enabling high reliability and low latency in future wireless networks.
@ARTICLE{Charan2021,
author={Charan, Gouranga and Alrabeiah, Muhammad and Alkhateeb, Ahmed},
journal={IEEE Transactions on Vehicular Technology},
title={Vision-Aided 6G Wireless Communications: Blockage Prediction and Proactive Handoff},
year={2021},
volume={70},
number={10},
pages={10193-10208},
doi={10.1109/TVT.2021.3104219}}
To reproduce the results in this paper:
Example: Steps to generate the results in this figure
- Coming soon
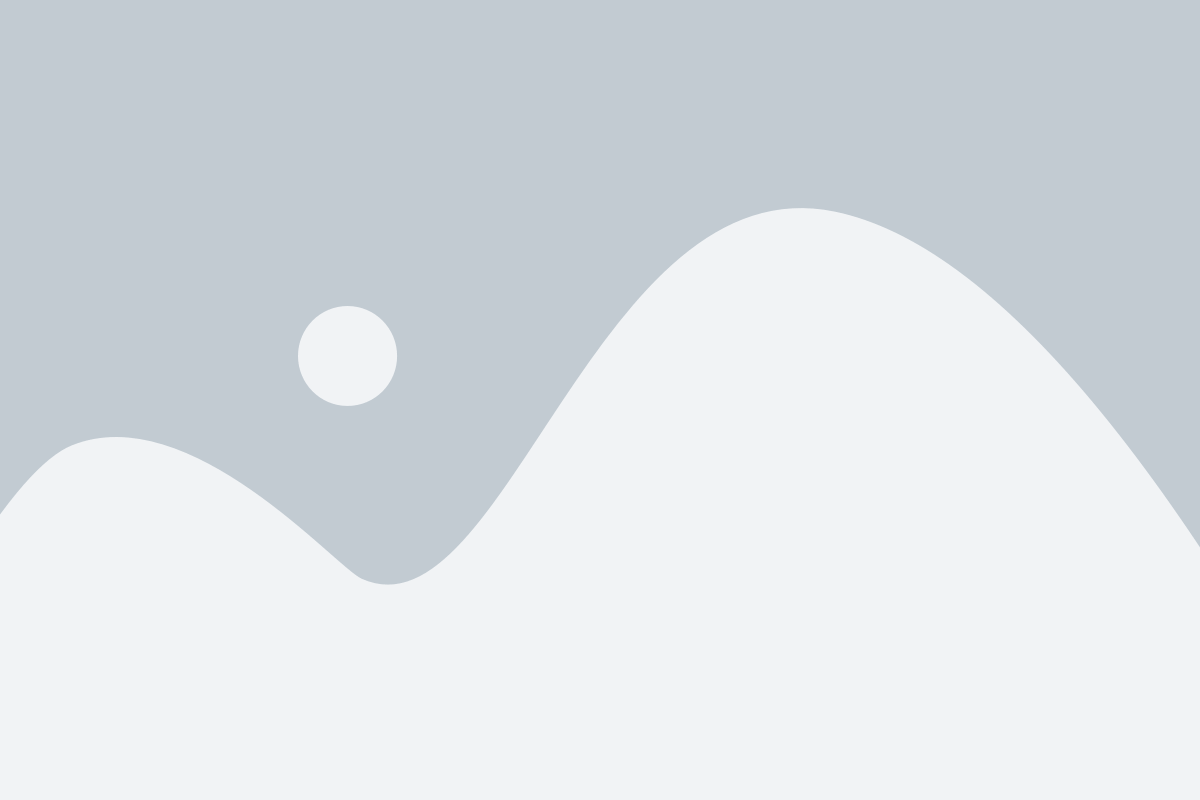
Having questions or feedback?
Send an email to