Towards Real-World 6G Drone Communication: Position and Camera Aided Beam Prediction
IEEE Global Communications Conference (GLOBECOM) 2022
Gouranga Charan, Andrew Hredzak, Christian Stoddard, Benjamin Berrey, Madhav Seth, Hector Nunez, Ahmed Alkhateeb
Wireless Intelligence Lab, ASU
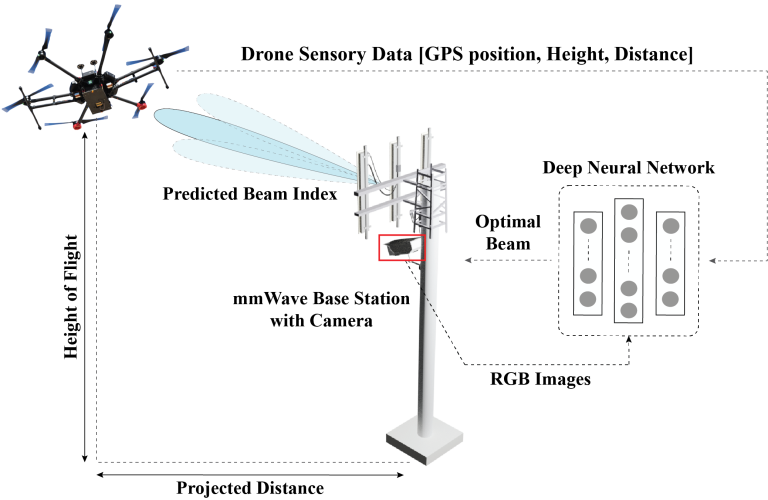
An illustration of the mmWave basestation serving a drone in a real wireless environment. The basestation utilizes additional sensing data such as RGB images, GPS location of the drone, etc., to predict the optimal beam.
Abstract
Millimeter-wave (mmWave) and terahertz (THz) communication systems typically deploy large antenna arrays to guarantee sufficient receive signal power. The beam training overhead associated with these arrays, however, make it hard for these systems to support highly-mobile applications such as drone communication. To overcome this challenge, this paper proposes a machine learning based approach that leverages additional sensory data, such as visual and positional data, for fast and accurate mmWave/THz beam prediction. The developed framework is evaluated on a real-world multi-modal mmWave drone communication dataset comprising co-existing camera, practical GPS, and mmWave beam training data. The proposed sensing-aided solution achieves a top-1 beam prediction accuracy of 86.32% and close to 100% top-3 and top-5 accuracies, while considerably reducing the beam training overhead. This highlights a promising solution for enabling highly-mobile 6G drone communications.
Proposed Solution
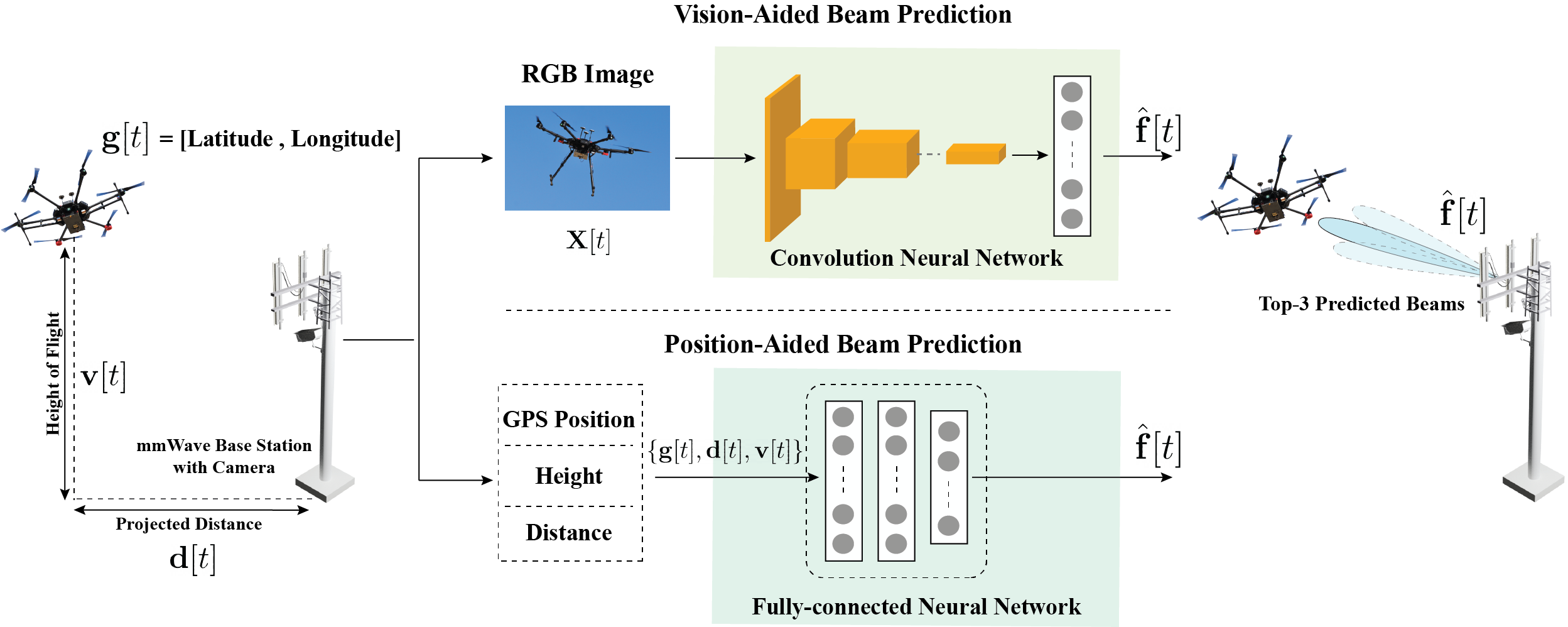
A block diagram showing the proposed solution for both the vision and position-aided beam prediction task. As shown in the figure, the camera installed at the basestation captures real-time images of the drone in the wireless environment. A CNN is then utilized to predict the optimal beam index. The basestation receives the information for the other three sensing data, which is then provided to a fully-connected neural network to predict the beam.
Video Presentation
Reproducing the Results
DeepSense 6G Dataset
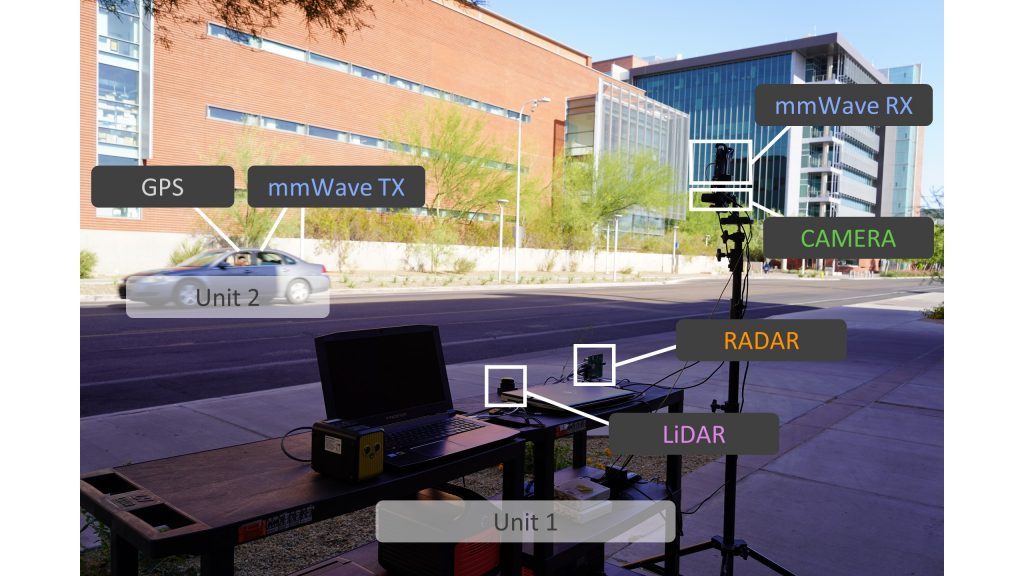
DeepSense 6G is a real-world multi-modal dataset that comprises coexisting multi-modal sensing and communication data, such as mmWave wireless communication, Camera, GPS data, LiDAR, and Radar, collected in realistic wireless environments. Link to the DeepSense 6G website is provided below.
Scenarios
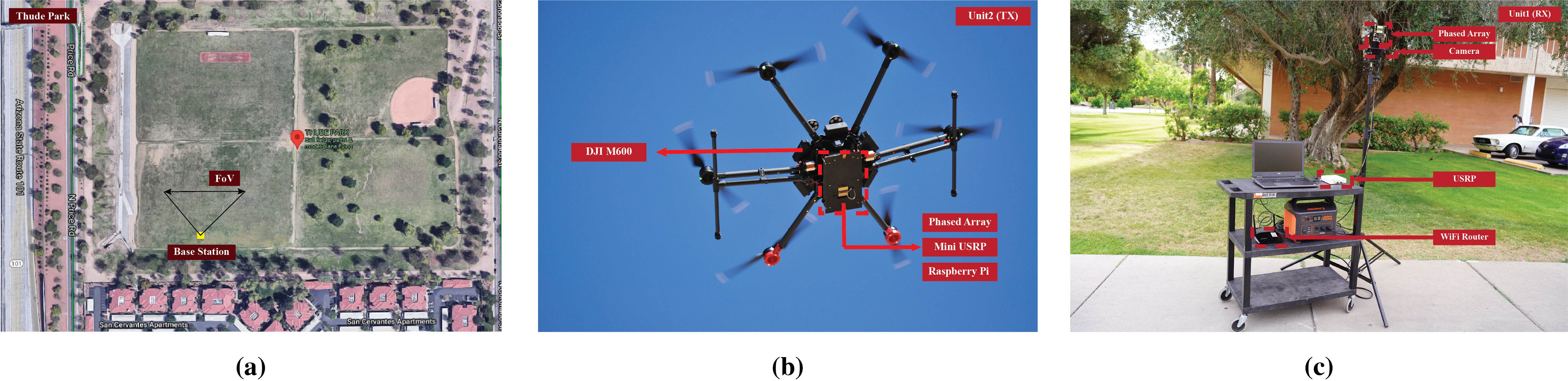
This figure presents the overview of the DeepSense 6G testbed and the location used in this scenario. (a) Shows the google map top-view of Thude Park utilized for this data collection. Fig. (b) and (c) present the different components of the drone (acting as the transmitter) and the basestation. In (b), we highlight the mmWave phased array attached to the drone transmitting signals to the basestation on the 60 GHz band.
Citation
A. Alkhateeb, G. Charan, T. Osman, A. Hredzak, J. Morais, U. Demirhan, and N. Srinivas, “DeepSense 6G: A Large-Scale Real-World Multi-Modal Sensing and Communication Datasets,” IEEE Communications Magazine, 2023.
@Article{DeepSense,
author={Alkhateeb, Ahmed and Charan, Gouranga and Osman, Tawfik and Hredzak, Andrew and Morais, Joao and Demirhan, Umut and Srinivas, Nikhil},
title={{DeepSense 6G: A Large-Scale Real-World Multi-Modal Sensing and Communication Dataset}},
journal={IEEE Communications Magazine},
year={2023},
publisher={IEEE}}
G. Charan, A. Hredzak, C. Stoddard, B. Berrey, M. Seth, H. Nunez, and A. Alkhateeb, “Towards Real-World 6G Drone Communication: Position and Camera Aided Beam Prediction”, In Proc. of IEEE Global Communications Conference (GLOBECOM), 2022
@INPROCEEDINGS{Charan2022,
title={Towards real-world {6G} drone communication: Position and camera aided beam prediction},
author={Charan, Gouranga and Hredzak, Andrew and Stoddard, Christian and Berrey, Benjamin and Seth, Madhav and Nunez, Hector and Alkhateeb, Ahmed},
booktitle={GLOBECOM 2022-2022 IEEE Global Communications Conference},
pages={2951–2956},
year={2022},
organization={IEEE}}