Camera Based mmWave Beam Prediction: Towards Multi-Candidate Real-World Scenarios
Available on ArXiv
Gouranga Charan, Muhammad Alrabeiah, Tawfik Osman, and Ahmed Alkhateeb
Wireless Intelligence Lab
Arizona State University
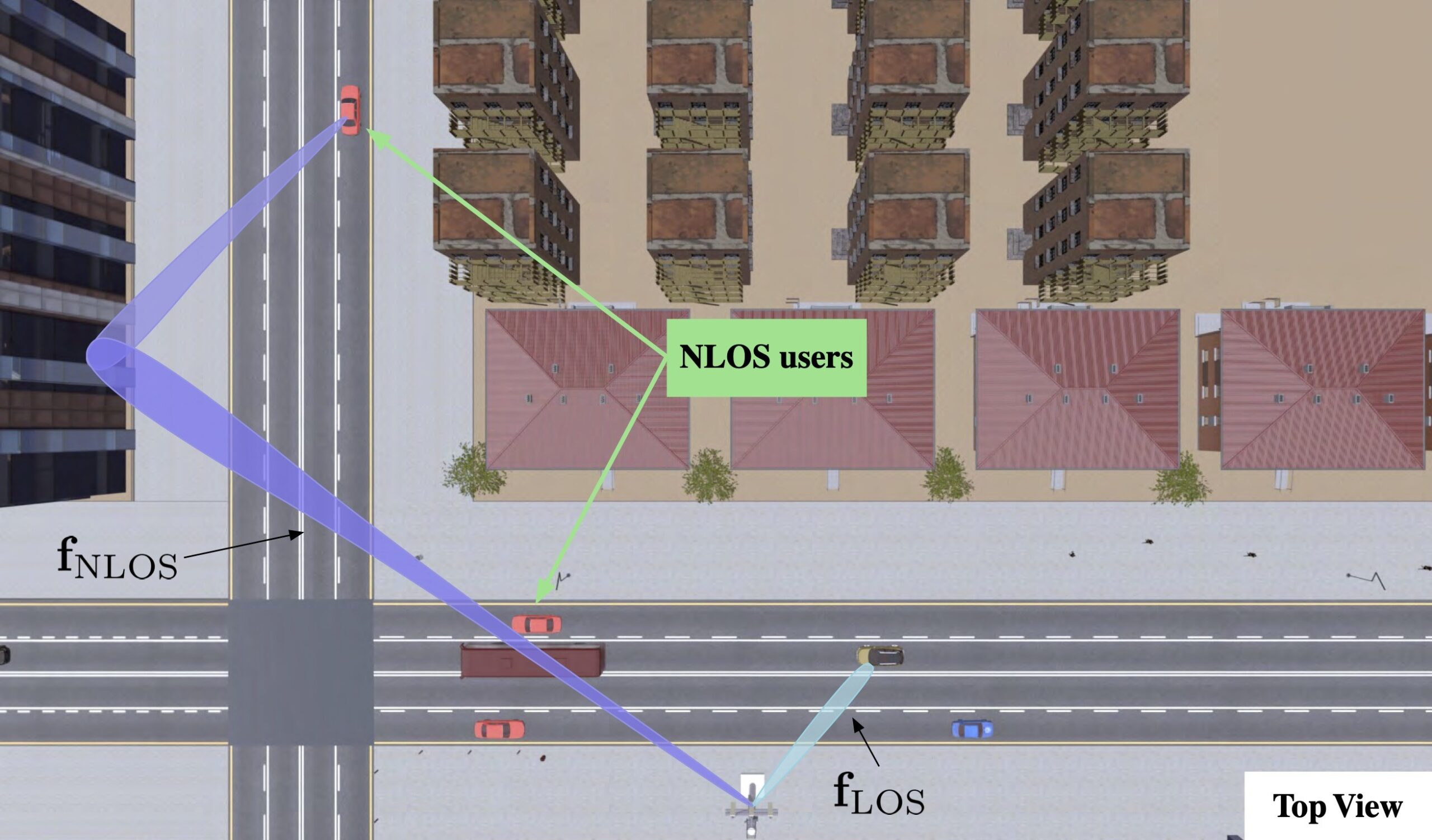
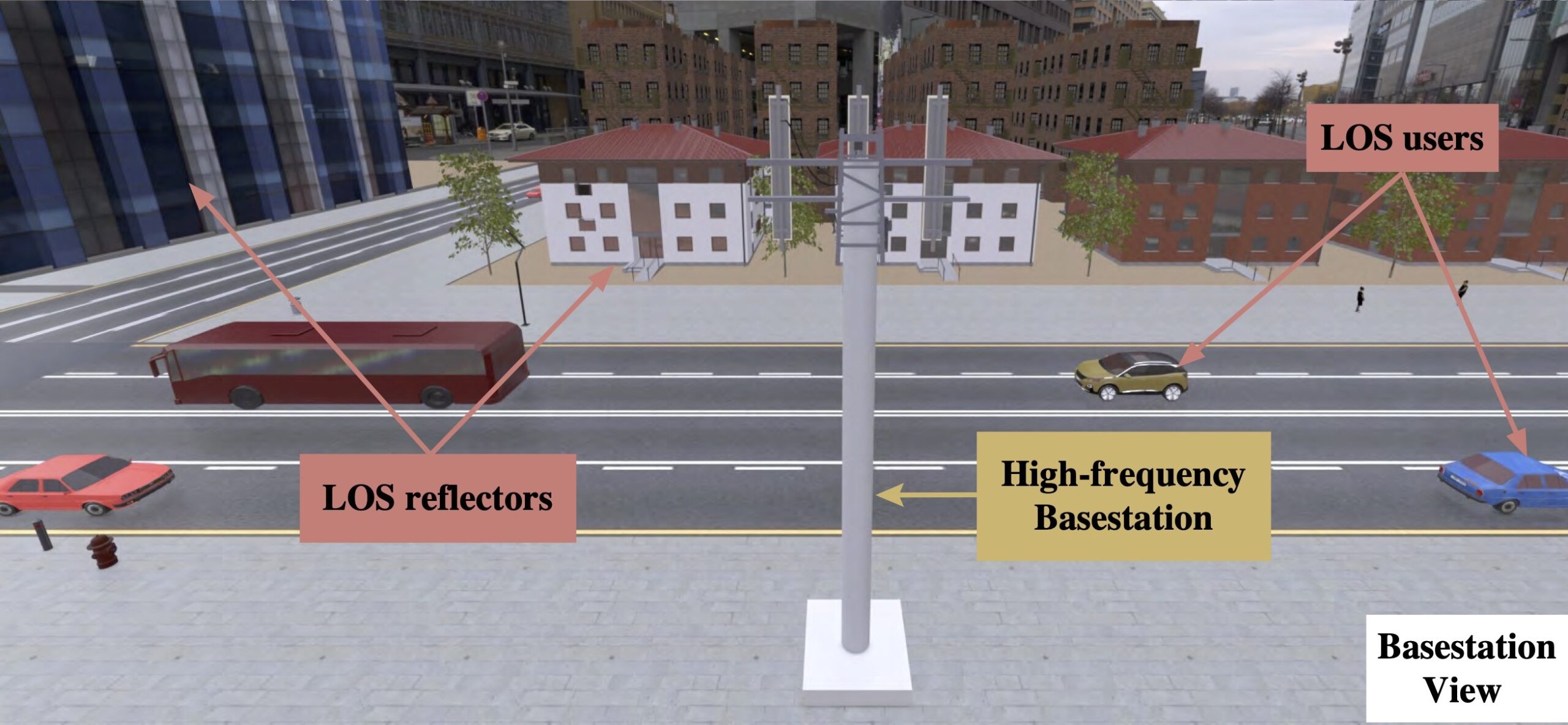
An illustration of a high-frequency wireless communication system and its environment. “Base station View” depicts the environment from the base station perspective, showing some LOS users and possible LOS reflectors. “Top View” shows the invisible part of that environment, i.e., NLOS users, and the beamforming vectors used to serve LOS and NLOS users.
Abstract
Leveraging sensory information to aid the millimeter-wave (mmWave) and sub-terahertz (sub-THz) beam selection process is attracting increasing interest. This sensory data, captured for example by cameras at the basestations, has the potential of significantly reducing the beam sweeping overhead and enabling highly-mobile applications. The solutions developed so far, however, have mainly considered single-candidate scenarios, i.e., scenarios with a single candidate user in the visual scene, and were evaluated using synthetic datasets. To address these limitations, this paper extensively investigates the sensing-aided beam prediction problem in a real-world multi-object vehicle-to-infrastructure (V2I) scenario and presents a comprehensive machine learning based framework. In particular, this paper proposes to utilize visual and positional data to predict the optimal beam indices as an alternative to the conventional beam sweeping approaches. For this, a novel user (transmitter) identification solution has been developed, a key step in realizing sensing-aided multi-candidate and multi-user beam prediction solutions. The proposed solutions are evaluated on the large-scale real-world DeepSense $6$G dataset. Experimental results in realistic V2I communication scenarios indicate that the proposed solutions achieve close to 100% top-5 beam prediction accuracy for the scenarios with single-user and close to 95% top-5 beam prediction accuracy for multi-candidate scenarios. Furthermore, the proposed approach can identify the probable transmitting candidate with more than 93% accuracy across the different scenarios. This highlights a promising approach for nearly eliminating the beam training overhead in mmWave/THz communication systems.
Proposed Solution
Single-Candidate Beam Prediction
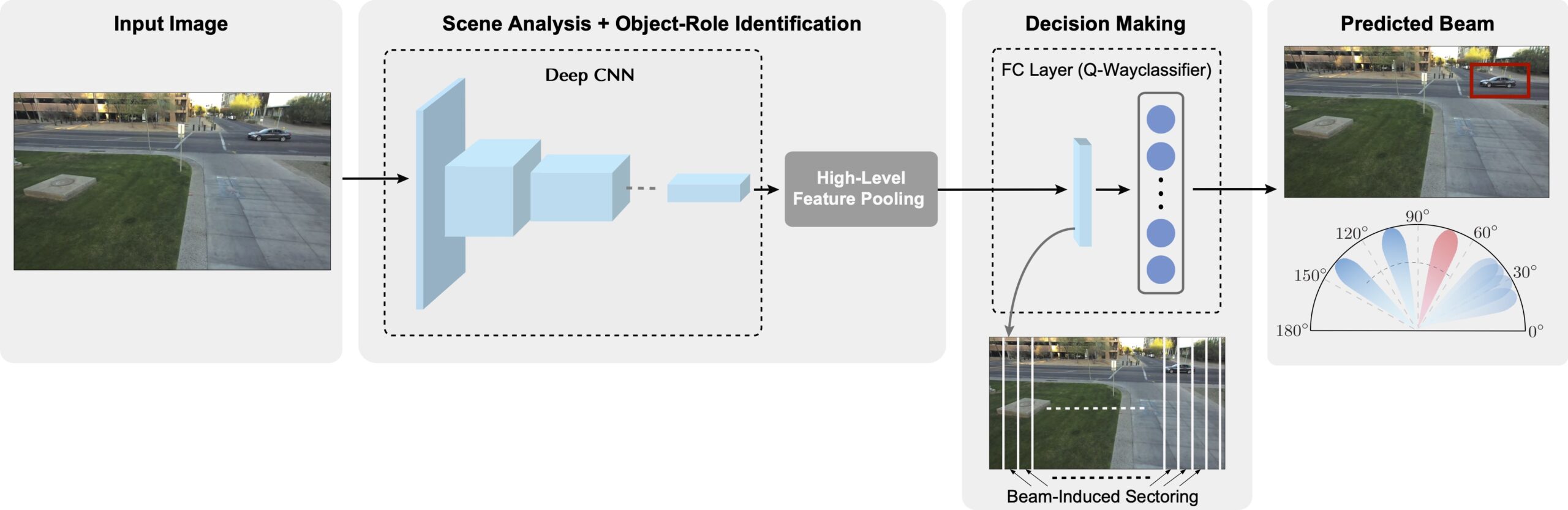
This figure illustrates the proposed machine learning-based vision-aided beam prediction model that leverages visual data captured at the base station for mmWave/sub-THz beam prediction in a single-candidate setting.
Multi-Candidate Beam Prediction
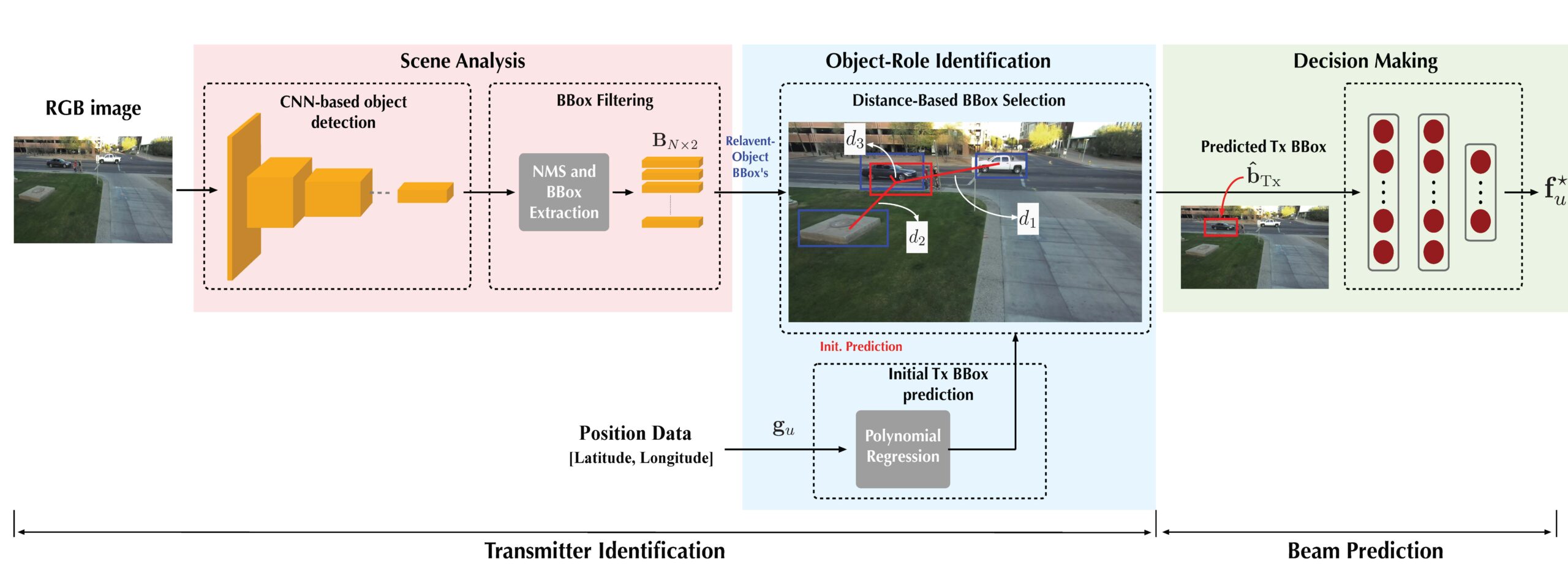
The figure presents the proposed multi-modal machine learning based beam prediction model that leverages both visual and positional data to predict the optimal beam indices in a multi-candidate settings.
Video Presentation
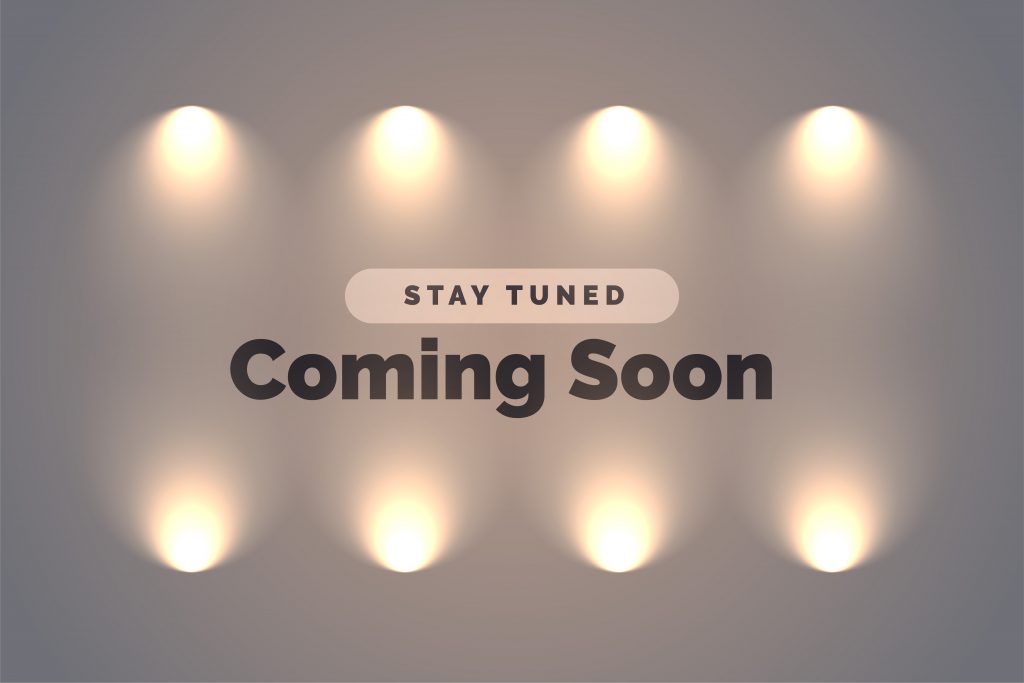
DeepSense 6G Dataset
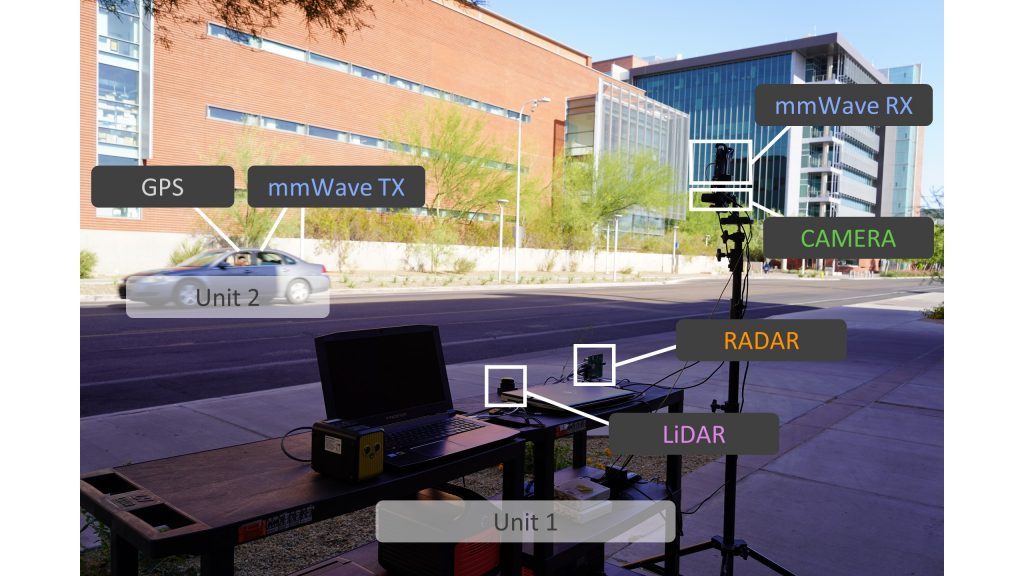
DeepSense 6G is a real-world multi-modal dataset that comprises coexisting multi-modal sensing and communication data, such as mmWave wireless communication, Camera, GPS data, LiDAR, and Radar, collected in realistic wireless environments. Link to the DeepSense 6G website is provided below.
Scenarios 1, 2, 3 and 4
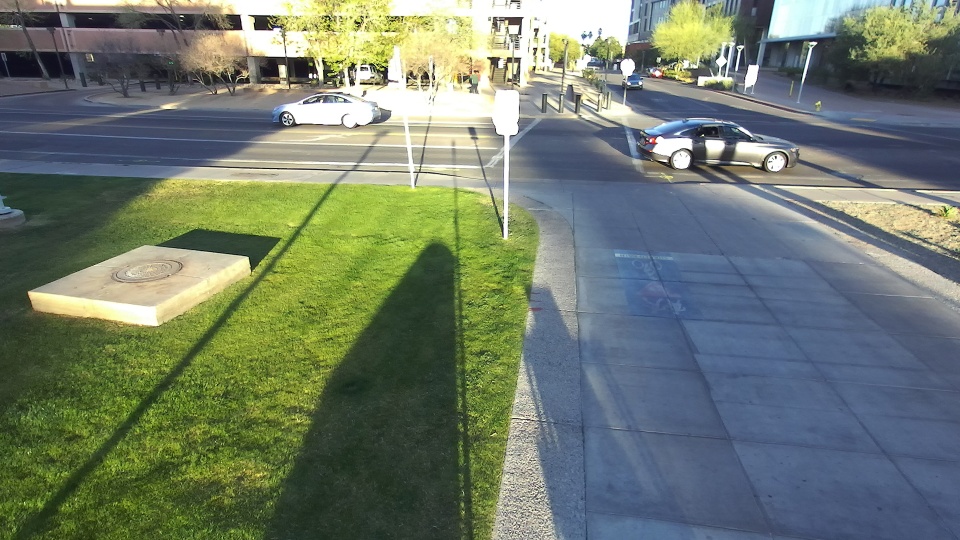
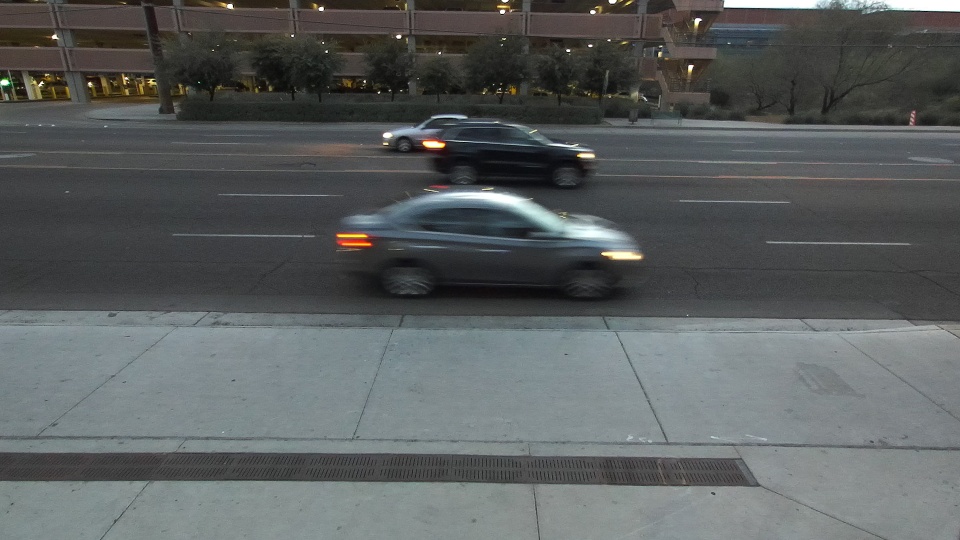
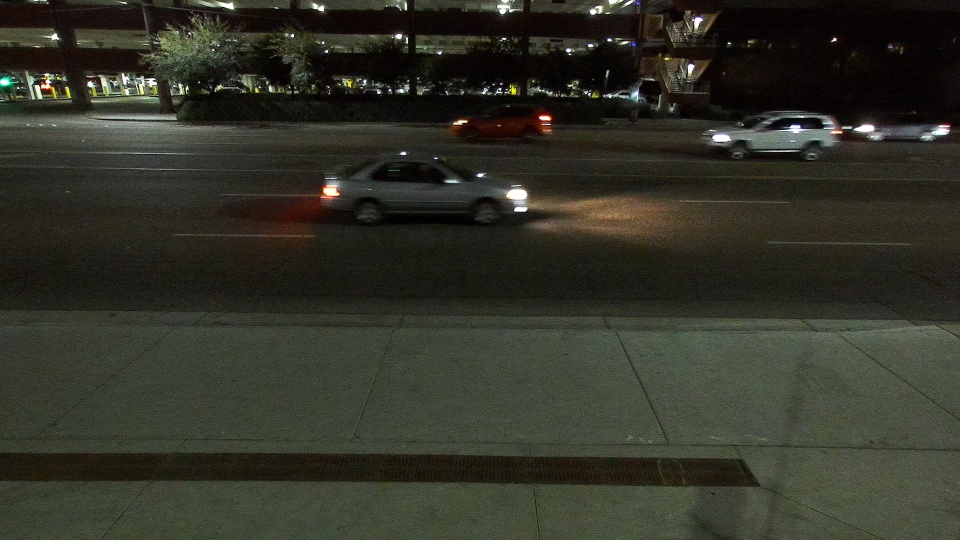
In this figure, we present the different image samples of scenarios 1, 2, 3, and 4. It shows the different lighting conditions (day, dusk, and night) in which the dataset was collected, highlighting the diversity in these scenarios.
Citation
A. Alkhateeb, G. Charan, T. Osman, A. Hredzak, J. Morais, U. Demirhan, and N. Srinivas, “DeepSense 6G: A Large-Scale Real-World Multi-Modal Sensing and Communication Datasets,” IEEE Communications Magazine, 2023.
@Article{DeepSense,
author={Alkhateeb, Ahmed and Charan, Gouranga and Osman, Tawfik and Hredzak, Andrew and Morais, Joao and Demirhan, Umut and Srinivas, Nikhil},
title={DeepSense 6G: A Large-Scale Real-World Multi-Modal Sensing and Communication Dataset},
journal={IEEE Communications Magazine},
year={2023},
publisher={IEEE}}
G. Charan, M. Alrabeiah, T. Osman, and A. Alkhateeb, “Camera Based mmWave Beam Prediction: Towards Multi-Candidate Real-World Scenarios” available on arXiv, 2023.
@Article{charan2023camera,
title={Camera Based mmWave Beam Prediction: Towards Multi-Candidate Real-World Scenarios},
author={Charan, Gouranga and Alrabeiah, Muhammad and Osman, Tawfik and Alkhateeb, Ahmed},
journal={arXiv preprint arXiv:2308.06868},
year={2023}}