Research
Aided Communication
Aided Communication
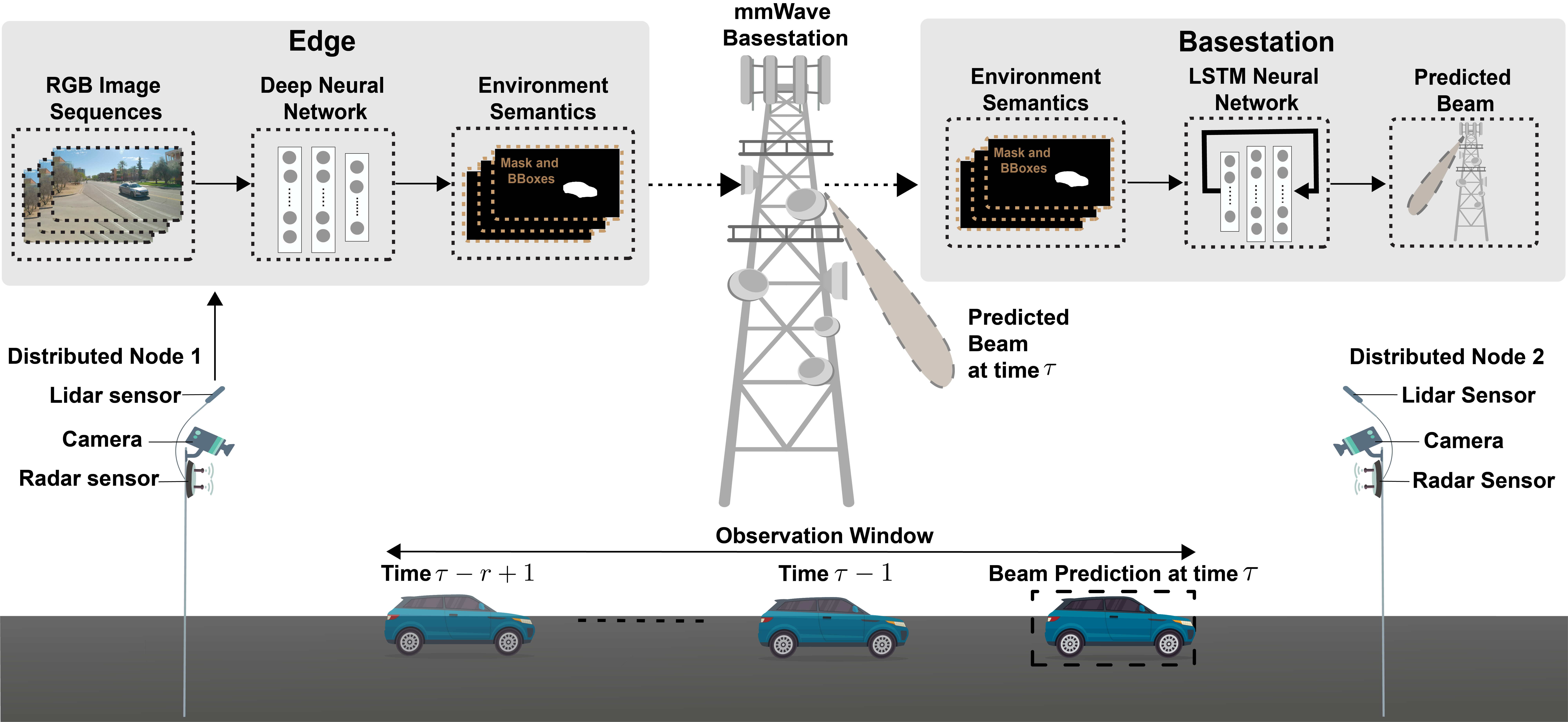
Environment Semantic Communication: Enabling Distributed Sensing Aided Networks
Millimeter-wave (mmWave) and terahertz (THz) communication systems require large antenna arrays and use narrow directive beams to ensure sufficient receive signal power. However, selecting the optimal beams for these large antenna arrays incurs a significant beam training overhead, making it challenging to support applications involving high mobility. … read more
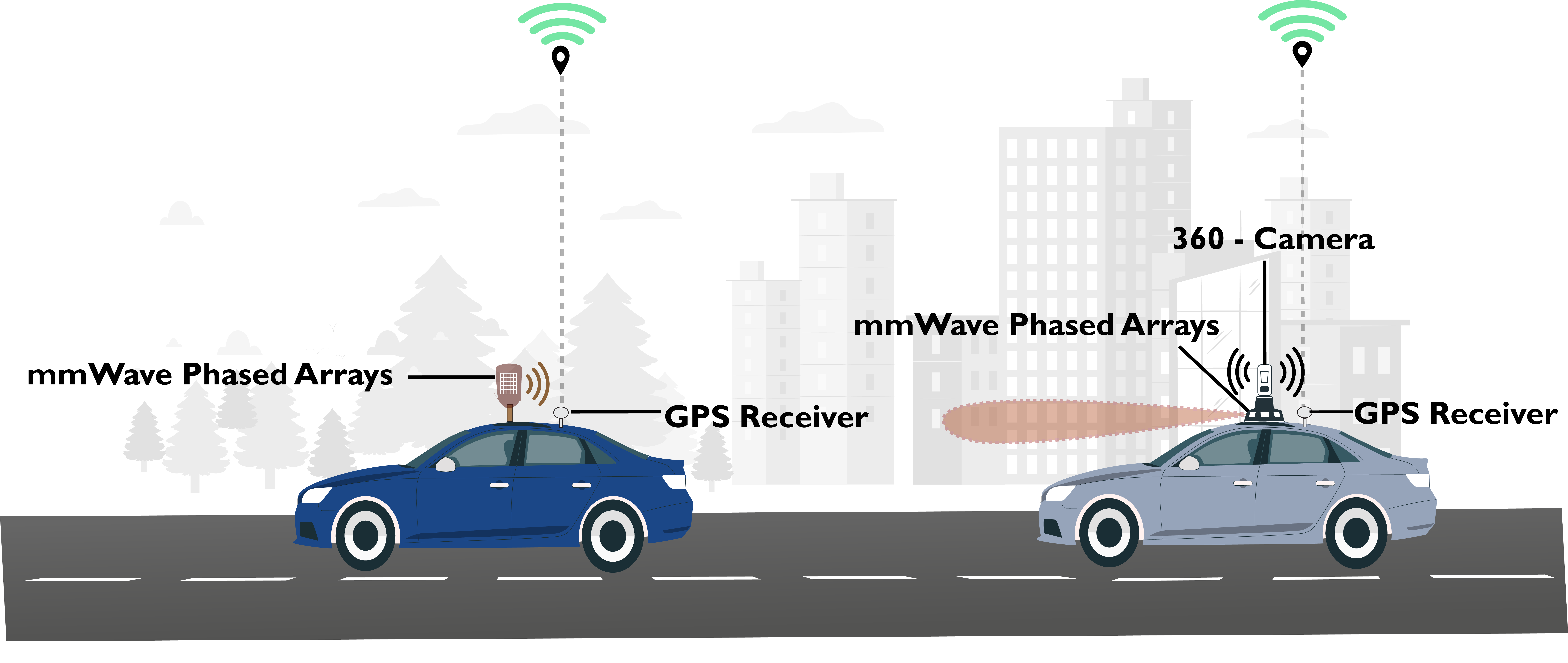
Vehicle Camera Guide mmWave Beams: Approach and Real-world V2V Demonstration
Accurately aligning millimeter-wave (mmWave) and terahertz (THz) narrow beams is essential to satisfy reliability and high data rates of 5G and beyond wireless communication systems. However, achieving this objective is difficult, especially in vehicle-to-vehicle (V2V) communication scenarios, where both transmitter and receiver are constantly mobile. Recently, additional sensing modalities, such as visual sensors, have attracted significant interest due to their capability to provide accurate information about the wireless environment. To that end, in this paper, we develop a deep learning solution for V2V scenarios to predict future beams using images from a 360 camera attached to the vehicle. … read more
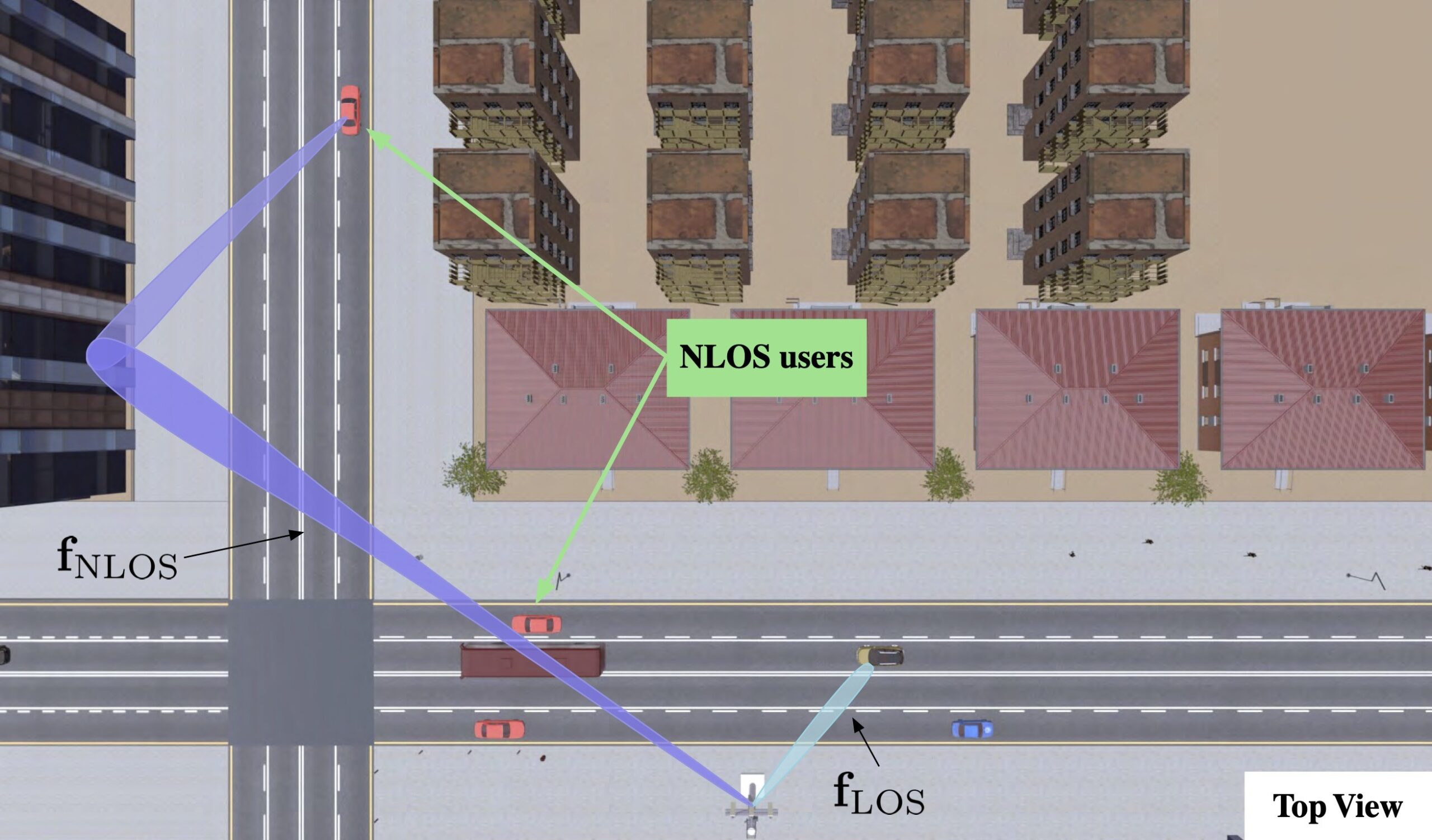
Camera Based mmWave Beam Prediction: Towards Multi-Candidate Real-World Scenarios
Leveraging sensory information to aid the millimeter-wave (mmWave) and sub-terahertz (sub-THz) beam selection process is attracting increasing interest. This sensory data, captured for example by cameras at the basestations, has the potential of significantly reducing the beam sweeping overhead and enabling highly-mobile applications. The solutions developed so far, however, have mainly considered single-candidate scenarios, i.e., scenarios with a single candidate user in the visual scene. … read more
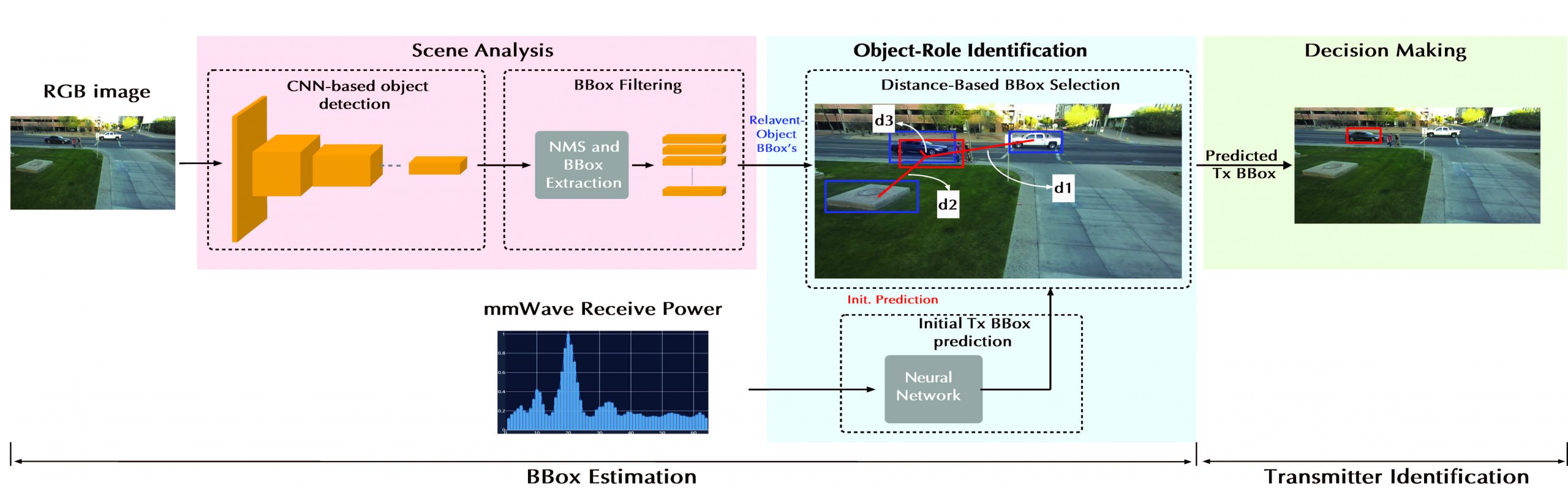
User Identification: The Key Enabler for Multi-User Vision-Aided Wireless Communications
Vision-aided wireless communication is attracting increasing interest and finding new use cases in various wireless communication applications. These vision-aided communication frameworks leverage the visual data captured, for example, by cameras installed at the infrastructure or mobile devices to construct some perception about the communication environment (geometry, users, scatterers, etc.). This is typically achieved through the use of deep learning and advances in computer vision and visual scene understanding. … read more
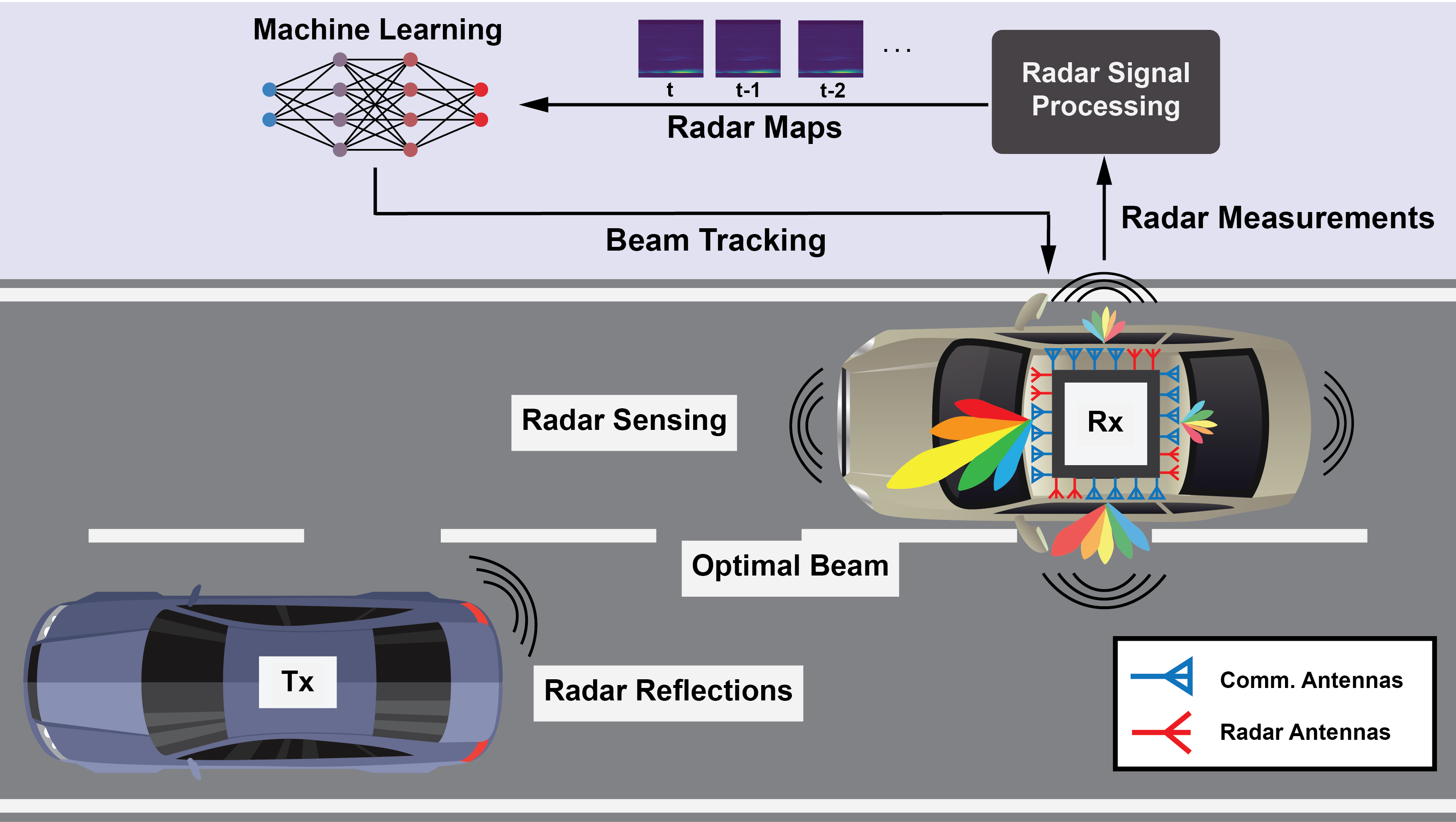
Millimeter Wave V2V Beam Tracking using Radar: Algorithms and Real-World Demonstration
Radar sensing applications for assisting communication have attracted interest thanks to their potential in dynamic environments. A particularly interesting problem for this approach appears in the vehicle-to-vehicle (V2V) millimeter wave and terahertz communication scenarios, where the narrow beams change with the movement of both vehicles. To address this problem, in this work, … read more
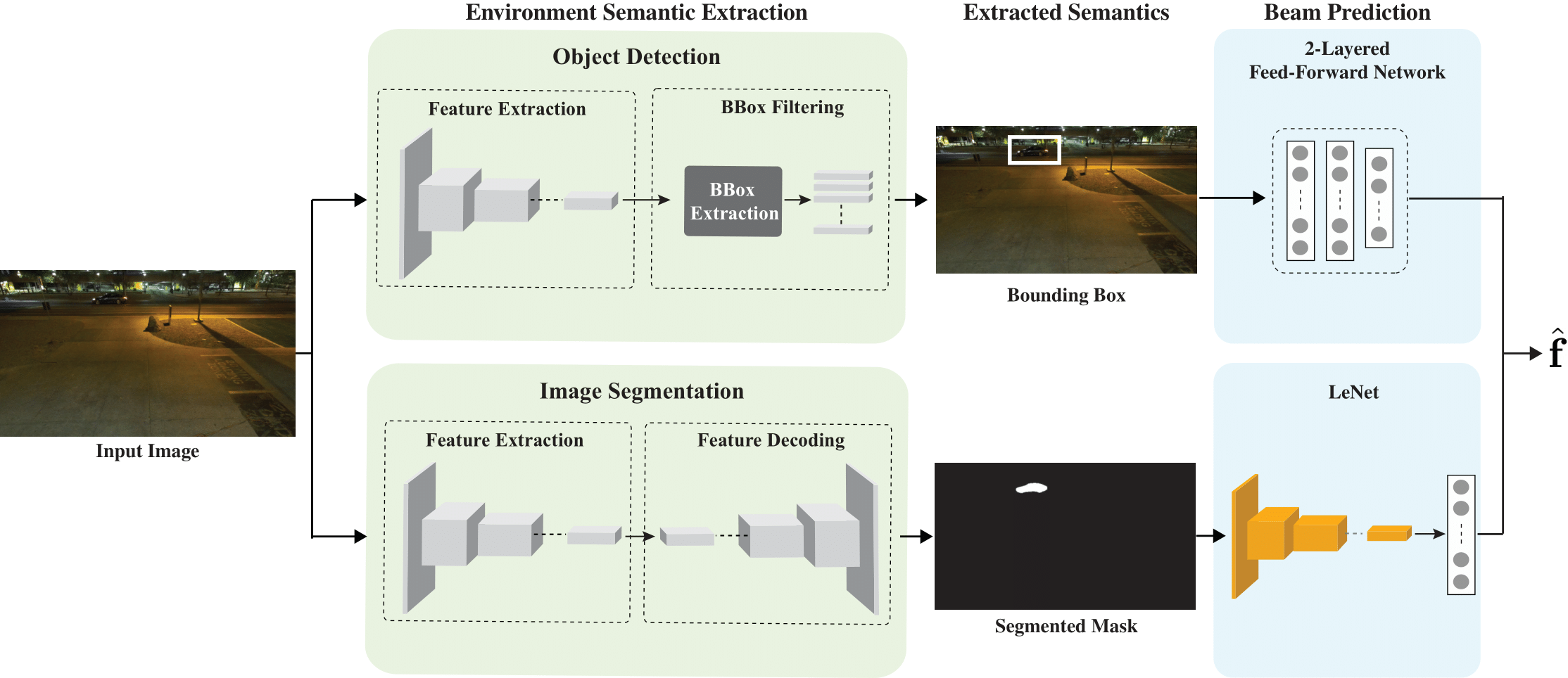
Environment Semantic Aided Communication: A Real World Demonstration for Beam Prediction
Millimeter-wave (mmWave) and terahertz (THz) communication systems adopt large antenna arrays to ensure adequate receive signal power. However, adjusting the narrow beams of these antenna arrays typically incurs high beam training overhead that scales with the number of antennas. Recently proposed vision-aided beam prediction solutions, which utilize raw RGB images captured at the basestation to predict the optimal beams, have shown initial promising results. However, they still have a considerable computational complexity, limiting their adoption in the real world. To address these challenges, … read more
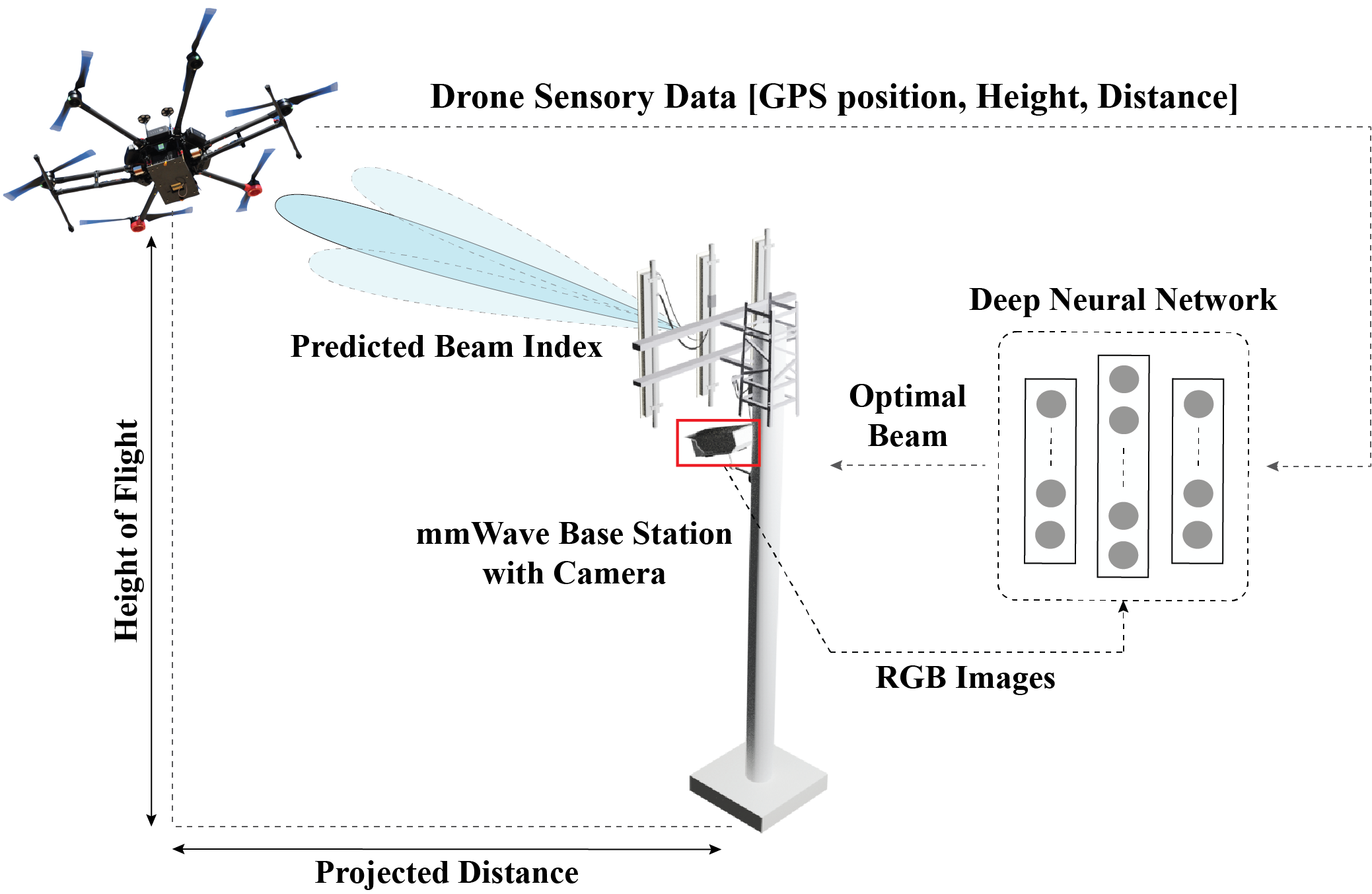
Towards Real-World 6G Drone Communication: Position and Camera Aided Beam Prediction
Millimeter-wave (mmWave) and terahertz (THz) communication systems typically deploy large antenna arrays to guarantee sufficient receive signal power. The beam training overhead associated with these arrays, however, make it hard for these systems to support highly-mobile applications such as drone communication. To overcome this challenge, this paper proposes a machine learning based approach that leverages additional sensory data, such as visual and positional data, for … read more
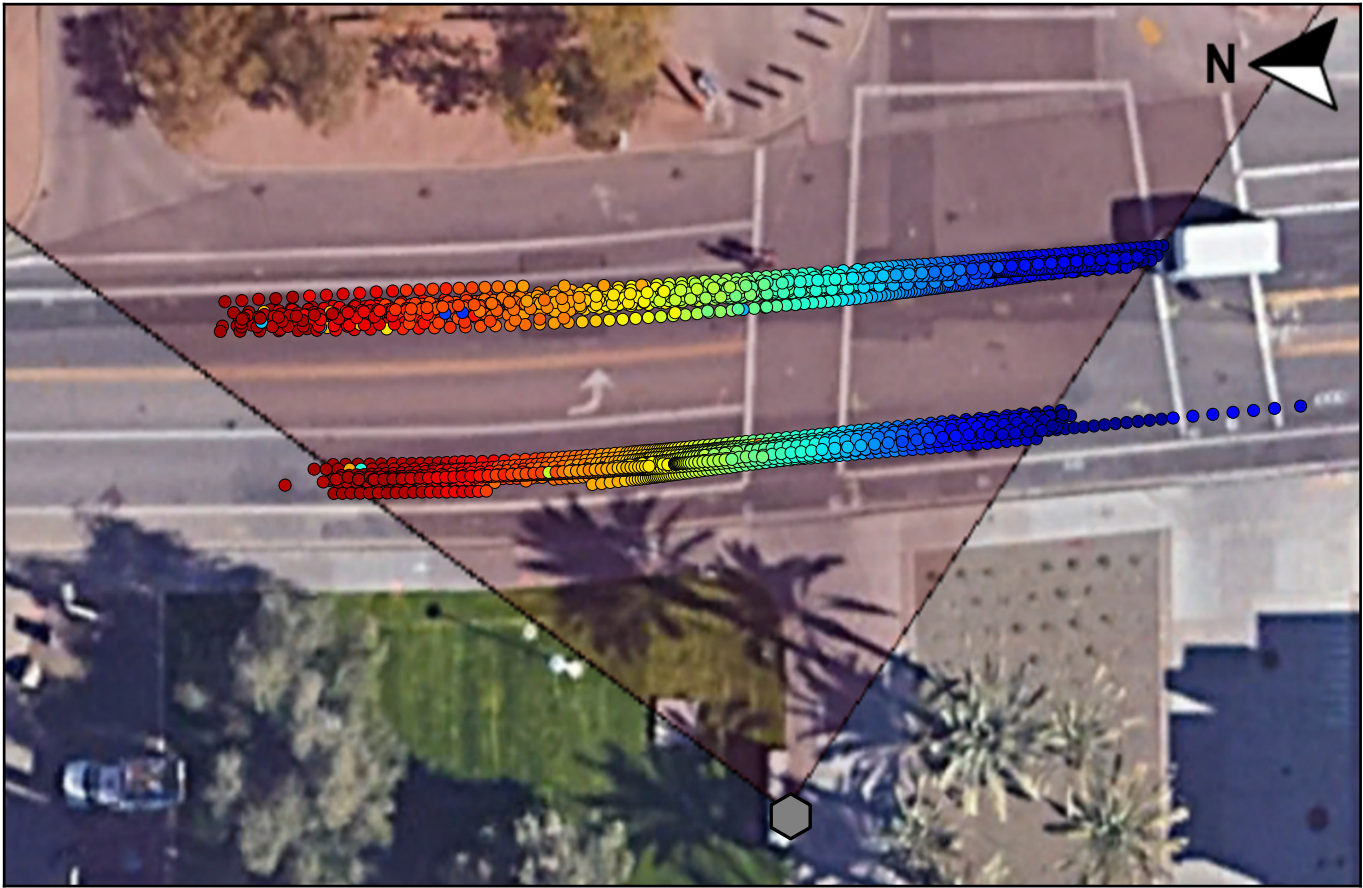
Position-aided Beam Prediction in the Real world: How useful GPS positions actually are?
Millimeter-wave communication systems rely on narrow beams for achieving sufficient receive signal power. Adjusting these beams is typically associated with large training overhead, which becomes particularly critical for highly mobile applications. Intuitively, since optimal beam selection can benefit from the knowledge of the positions of communication terminals, there has been increasing interest in leveraging position data to reduce the overhead in mmWave beam prediction. Prior work, however, studied this problem using only synthetic data that generally does not accurately represent real-world measurements. In this paper, we investigate position-aided beam prediction … read more
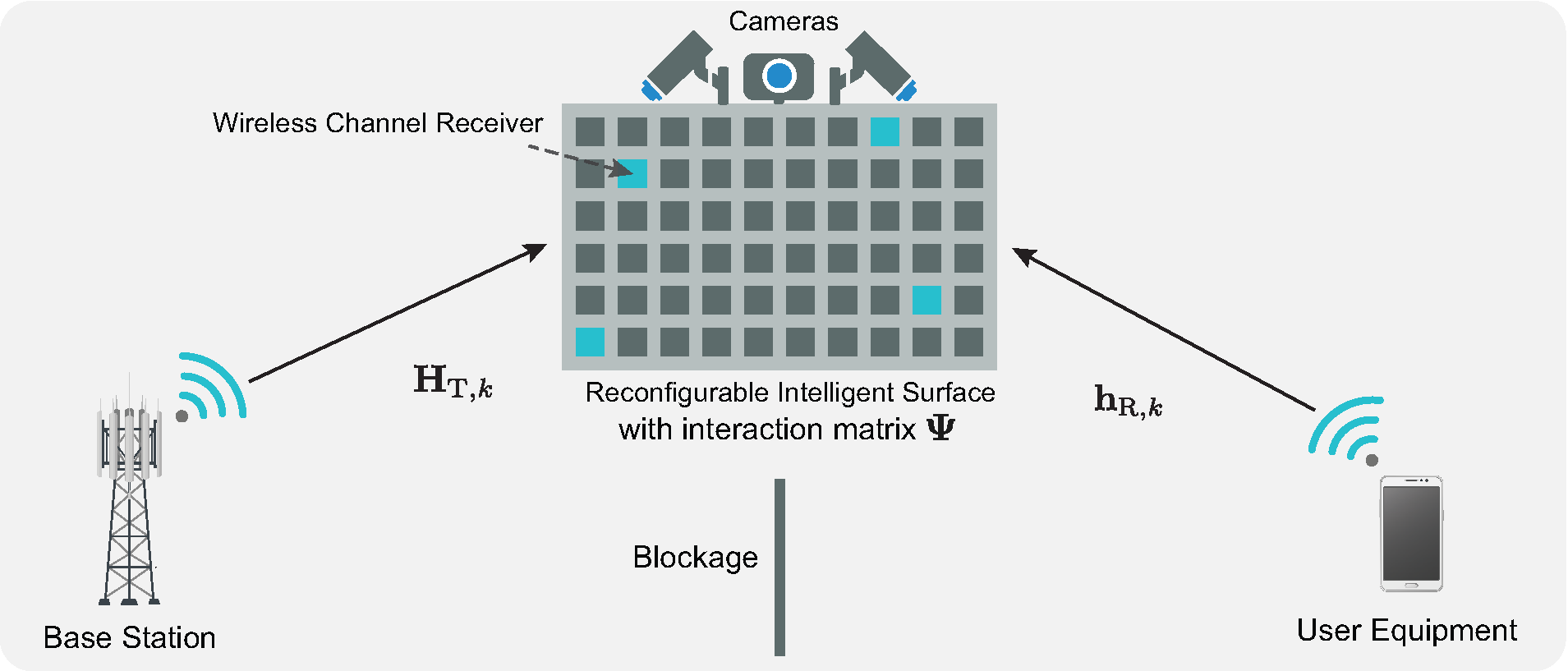
Sensing Aided Reconfigurable Intelligent Surfaces for 3GPP 5G Transparent Operation
This paper studies the feasibility of transparent RIS systems and shows that a key challenge lies in determining the user side RIS beam reflection direction. To address this challenge, the RIS leverages multi-modal sensing to develop perception of the surrounding environment and the mobile users. A ML framework then leverages the sensing information to select the high-performance beams and enable transparent RIS operation for 5G high-frequency systems … read more
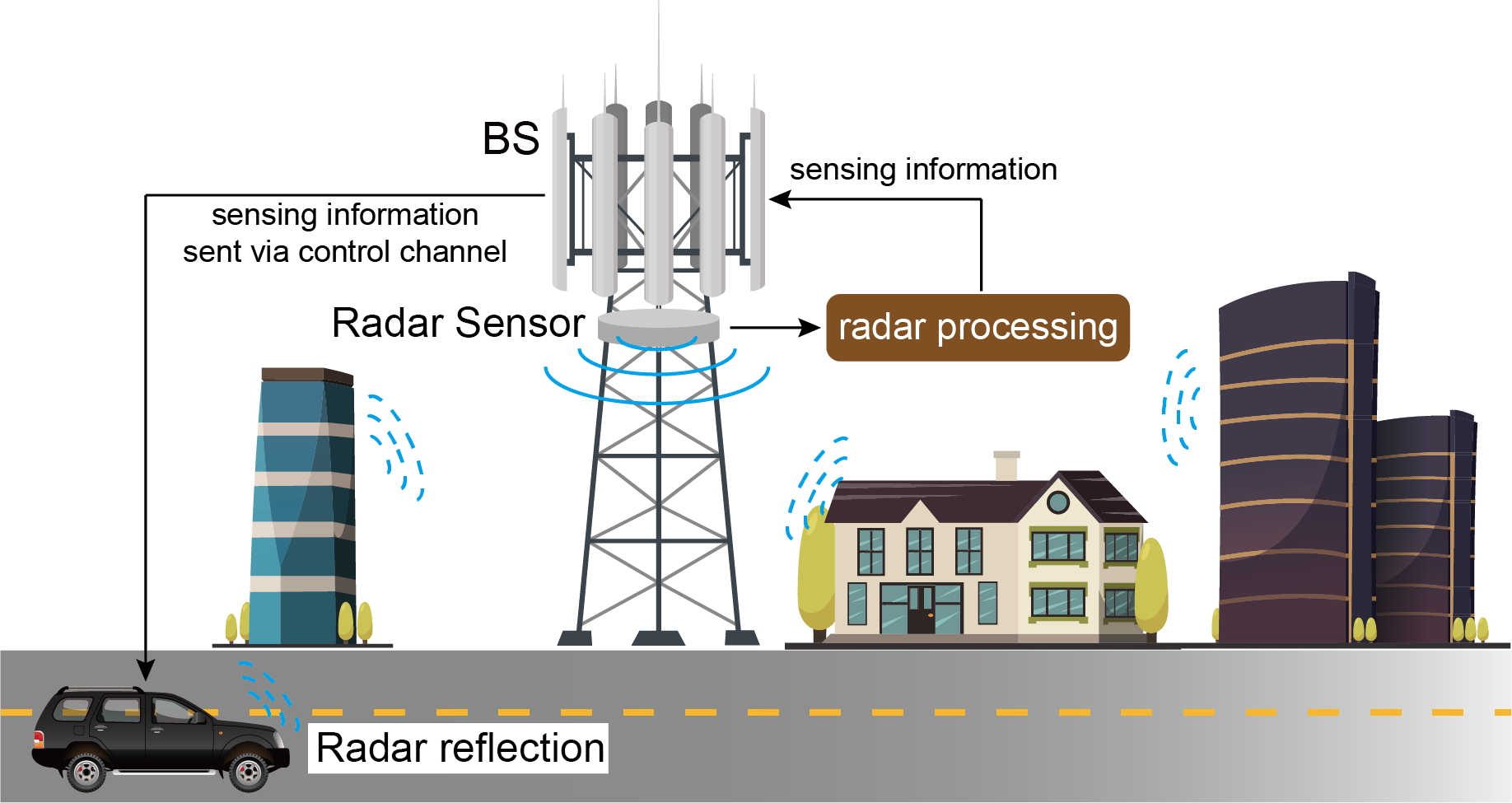
Sensing Aided OTFS Channel Estimation for Massive MIMO Systems
An important observation in OTFS system is that the delay, Doppler, and angle of departure/arrival information are directly related to the distance, velocity, and direction information of the mobile user and the various scatterers in the environment. We propose to leverage radar sensing to obtain this information about the mobile users and scatterers in the environment and leverage it to aid the OTFS channel estimation in massive MIMO systems … read more
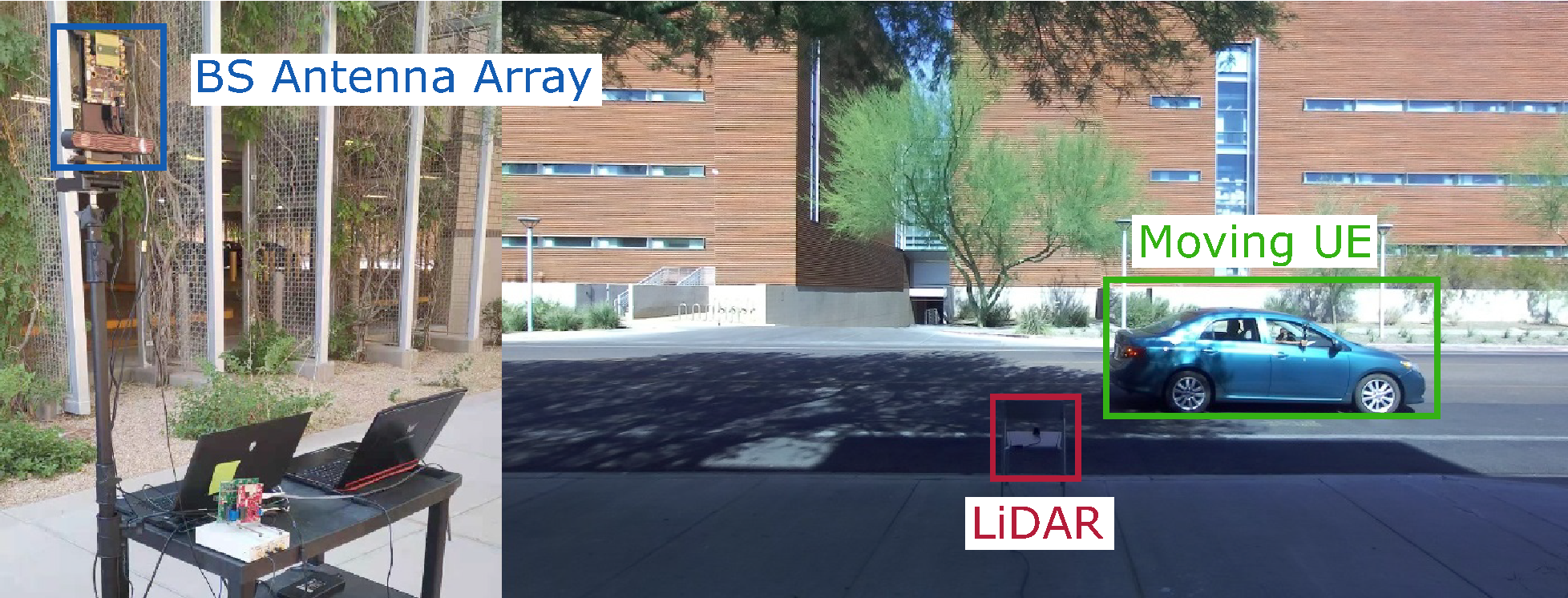
LiDAR Aided Future Beam Prediction in Real-World Millimeter Wave V2I Communications
This paper presents the first large-scale real-world evaluation for using LiDAR data to guide the mmWave beam prediction task. A machine learning (ML) model that leverages the LiDAR sensory data to predict the current and future beams was developed. Based on the large-scale real-world dataset, DeepSense 6G, this model was evaluated in a vehicle-to-infrastructure communication scenario with highly-mobile vehicles … read more
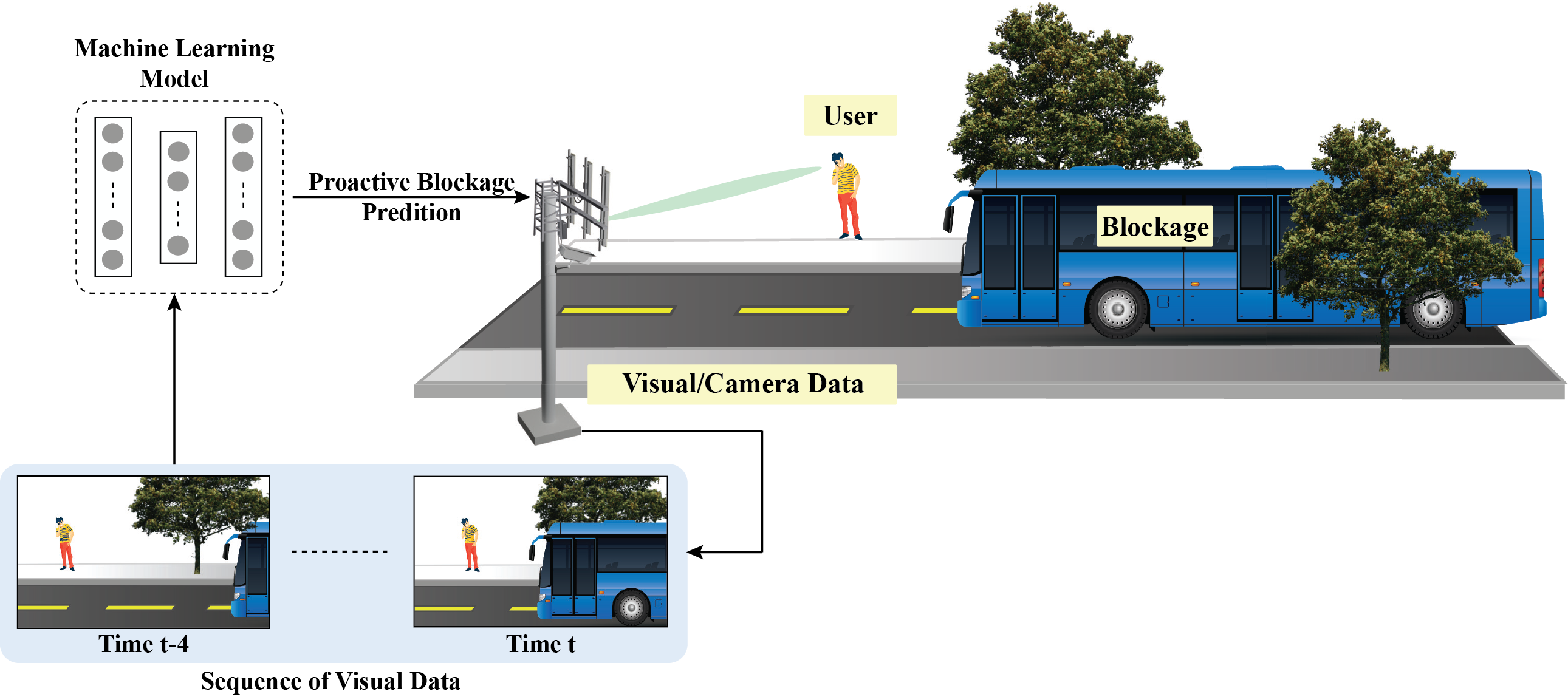
Computer Vision Aided Blockage Prediction in Real-World Millimeter Wave Deployments
This paper provides the first real-world evaluation of using visual (RGB camera) data and machine learning for proactively predicting millimeter wave (mmWave) dynamic link blockages before they happen. Proactively predicting line-of-sight (LOS) link blockages enables mmWave/sub-THz networks to make proactive network management decisions, such as proactive beam switching and hand-off) before a link failure happens. This can significantly enhance the network reliability and latency while efficiently utilizing the wireless resources. To evaluate this gain in reality, this paper … read more
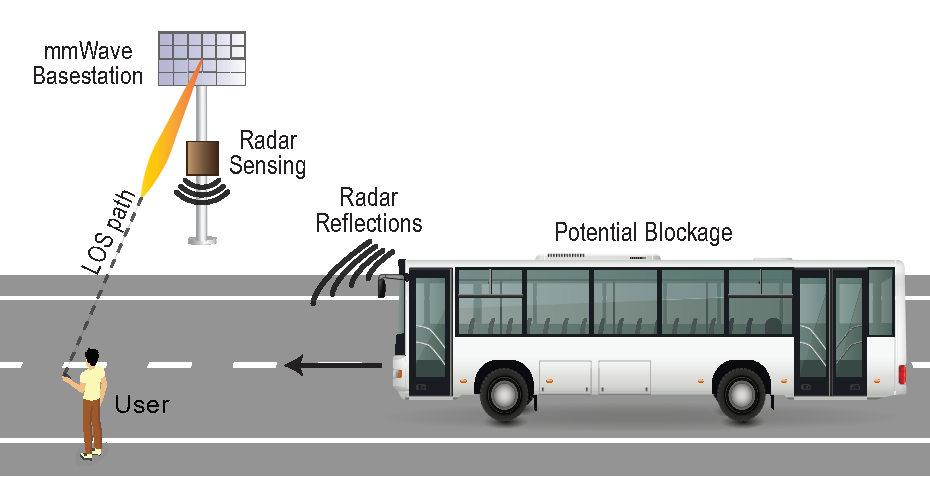
Radar Aided Proactive Blockage Prediction in Real-World Millimeter Wave Systems
In this paper, we propose to utilize radar sensors to
provide sensing information about the surrounding environment
and moving objects, and leverage this information to proactively
predict future link blockages before they happen … read more
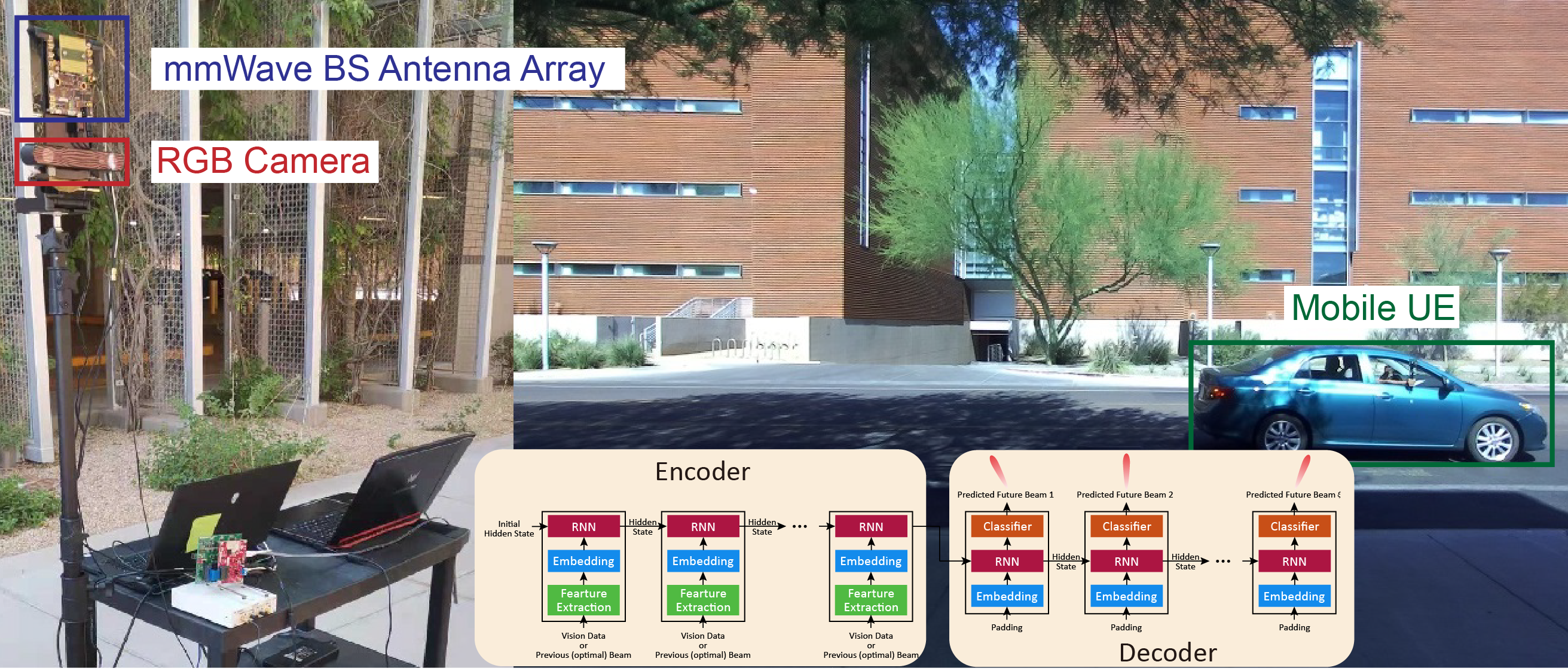
Computer Vision Aided Beam Tracking in A Real-World Millimeter Wave Deployment
This paper utilizes visual sensing information to enable fast and low-overhead mmWave/THz beam tracking. In this paper, we first propose a universal problem formulation for the auxiliary data-aided beam tracking, which could be used for different auxiliary data modalities. Then we propose a deep learning model for vision-aided mmWave/THz beam tracking exploiting an encoder-decoder architecture. The proposed vision-aided beam tracking is evaluated on the DeepSense real-world dataset … read more
Radar Aided 6G Beam Prediction: DL Algorithms and Real-World Demonstration
This paper presents the first machine learning based real-world demonstration for radar-aided beam prediction in a practical vehicular communication scenario. Leveraging radar sensory data at the communication terminals provides important awareness about the transmitter/receiver locations and the surrounding environment, which is leveraged for selecting the beams … read more
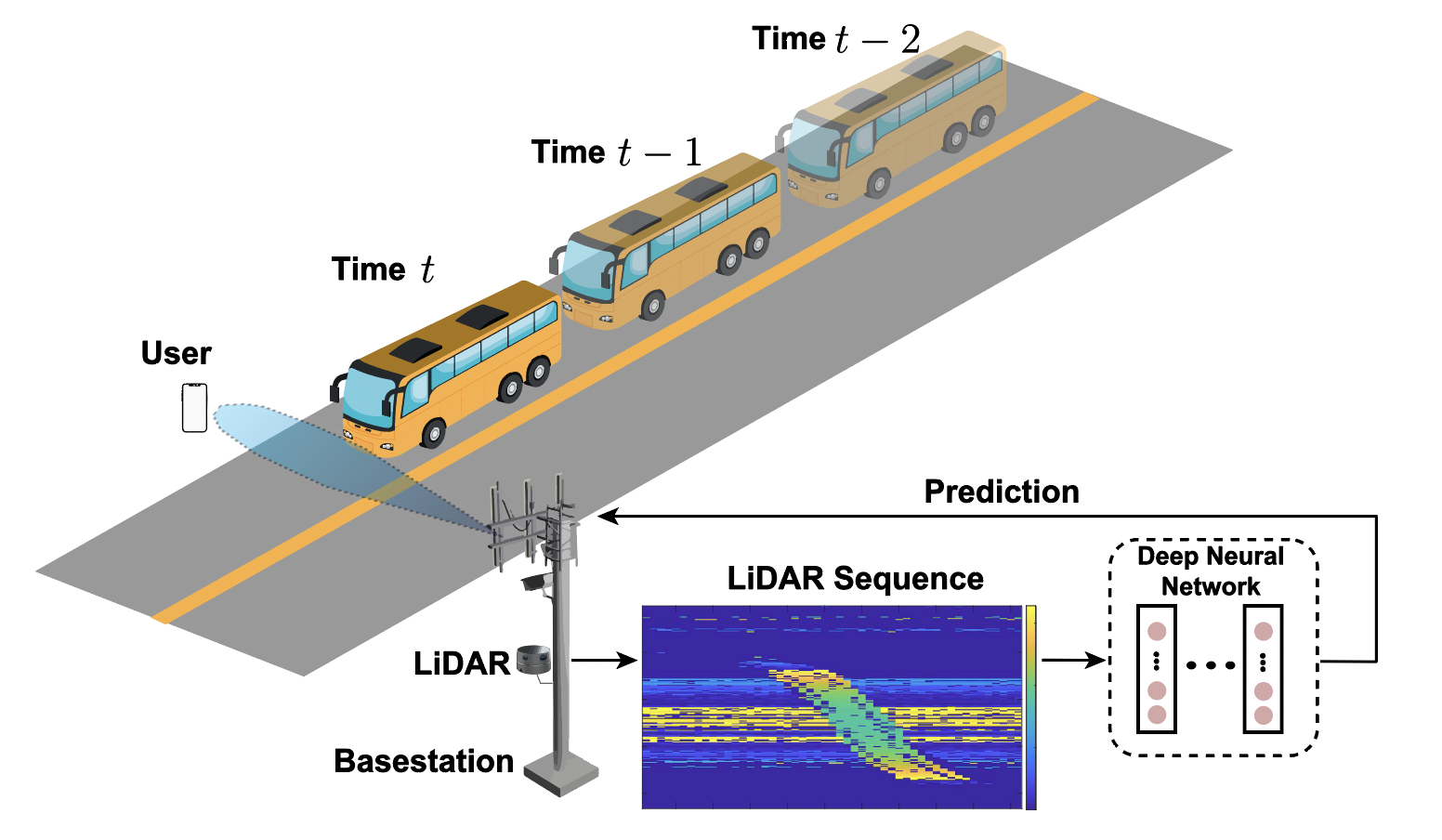
LiDAR-Aided Mobile Blockage Prediction in Real-World Millimeter Wave Systems
A typical LiDAR sensor sends pulsed light waves into the surrounding environment. These pulses are reflected by the objects and returned to the sensor; and the sensor uses the round-trip time to calculate the distance it traveled. By sending and receiving laser beams, the LiDAR sensor collects a 2-D point cloud map of the surrounding environment. We propose to leverage these LiDAR sensory data to detect if an object is going to block the mmWave communication link between the basestation and the mobile user. This figure shows an example when a communication link is getting blocked by a moving object … read more
Blockage Prediction Using Wireless Signatures in a Real-World Demonstration
The blockage prediction approaches in this paper are based on the following simple observation: Consider a fixed TX and RX in a certain environment with a LOS path. If an object moves in this environment till it blocks this LOS path, then, during the movement, the object acts like a scatterer for the signal propagating from the TX to the RX. The received signal during this interval will experience a constructive and destructive interference from the LOS ray and the ray scattered on the moving object. … read more
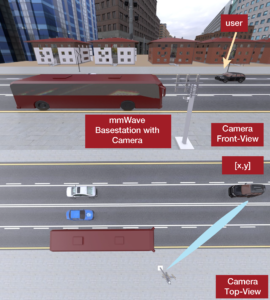
Vision-Position Multi-Modal Beam Prediction Using Real mmWave Datasets
One of the significant challenges associated with mmWave/THz communication systems is the large beam training overhead, especially for high-mobility scenarios. The interesting question here is, can additional sensory data such as data captured from the position and visual (RGB camera) sensors be utilized to reduce the beam training overhead and accurately predict the mmWave beams in 5G/B5G systems? This work attempts to answer this question using a large real-world diverse (different locations, scenarios, etc.) dataset in Vehicle-to-Infrastructure (V2I) mmWave communication scenarios. … read more
Computer Vision Aided URLL Communications
We propose a novel framework termed proactive service identification that utilizes visual data (captured by RGB cameras) and deep learning. The ultimate objective of this framework is to equip future wireless networks with the ability to analyze user behavior, anticipate incoming services, and perform proactive resource allocation … read more
Blockage Prediction and Proactive Handoff
We propose a novel machine learning based solution to tackle the reliability and latency challenges in mmWave MIMO systems. Simulation results show that the developed deep learning based strategy successfully predicts blockage/hand-off in close to 95% of the times … read more
Vision-Aided 6G Wireless Communications
One of the key challenges in mmWave/THz system arises from the sensitivity of the high-frequency signals to blockages. Sudden link blockages highly threaten the reliability of the networks and incur critical network latency. A promising way to tackle the reliability and latency challenges lies in enabling proaction in wireless networks. Proaction basically allows the network to anticipate blockages, especially dynamic blockages, and initiate user hand-off beforehand. In order to enable proaction, the network needs to first develop a sense of the wireless environment. … read more
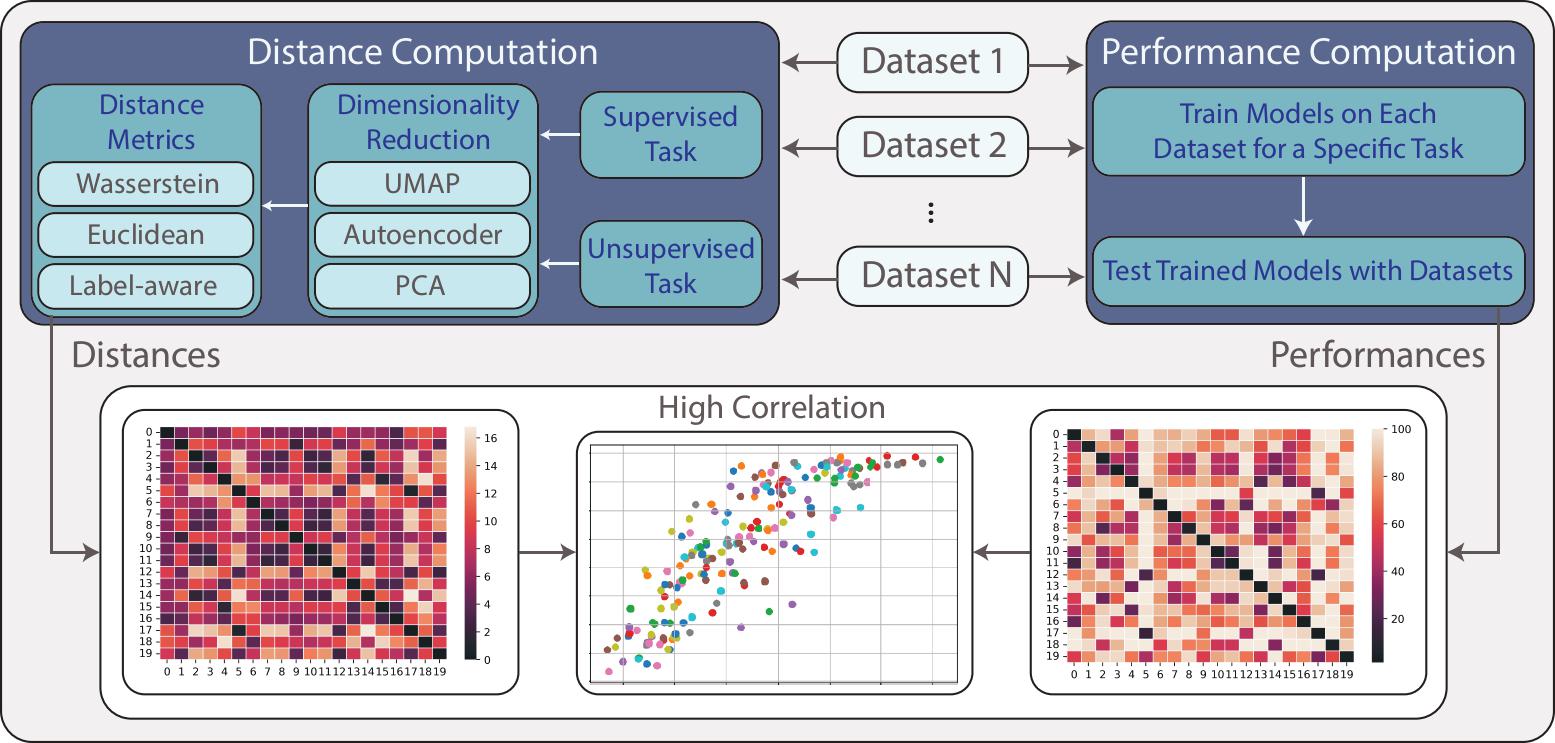
A Dataset Similarity Evaluation Framework for Wireless Communications and Sensing
Given the critical discrepancies between synthetic and real-world datasets, there is a pressing need to quantify these differences and how they affect model performance to ensure effective generalization and reliable performance in real world deployments. This paper proposes a task-specific, model-agnostic metric for measuring ‘dataset distance’ and effectively assess and compare the realism and quality of datasets. Such a metric would enable real-world data augmentation, facilitate effective benchmarking, and help in retraining decisions. … read more
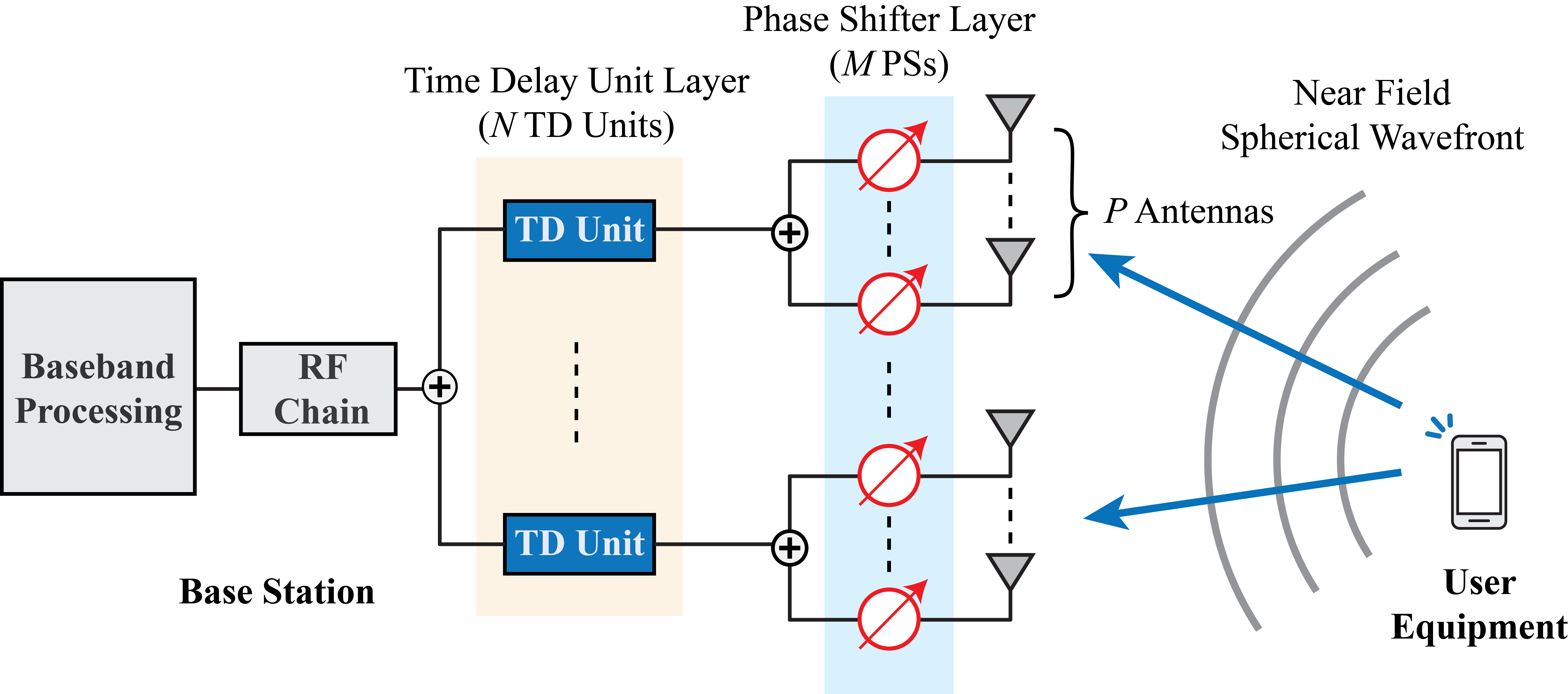
Beam Focusing for Wideband Near Field Massive MIMO Systems
We propose a low-complexity frequency-aware beamforming solution that is designed for hybrid time-delay and phase-shifter based RF architectures. To reduce the complexity, the joint design problem of the time delays and phase shifts is decomposed into two subproblems, where a signal model inspired online learning framework is proposed to learn the shifts of the quantized analog phase shifters, and a low-complexity geometry-assisted method is leveraged to configure the delay settings of the time-delay units … read more
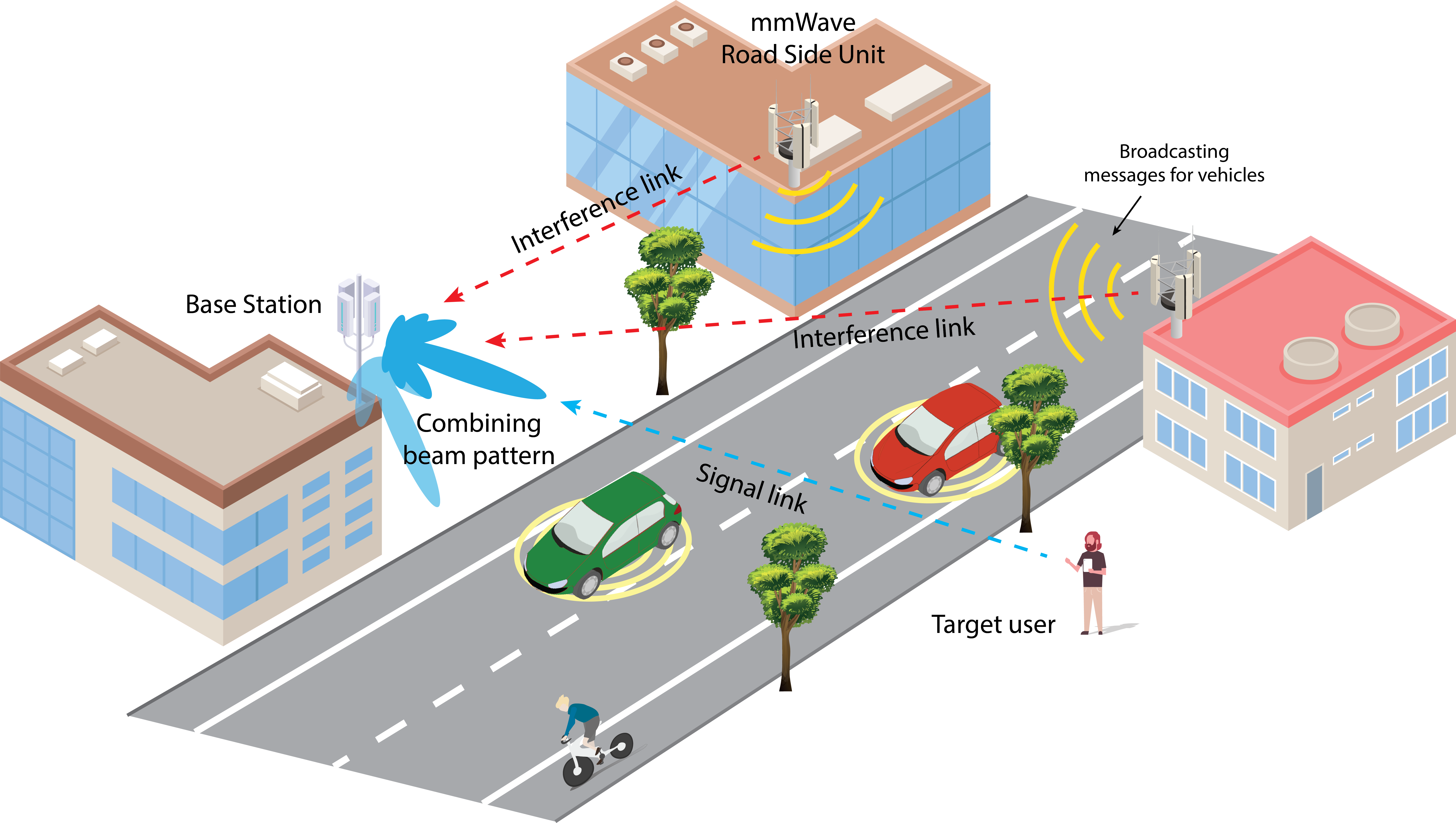
Interference Nulling in Hardware-Constrained mmWave MIMO Systems
We propose an efficient deep reinforcement learning approach that learns how to iteratively optimize the beam pattern to null the interference. The proposed solution achieves that while not requiring any explicit channel knowledge of the desired or interfering users and without requiring any coordination with the interferers … read more
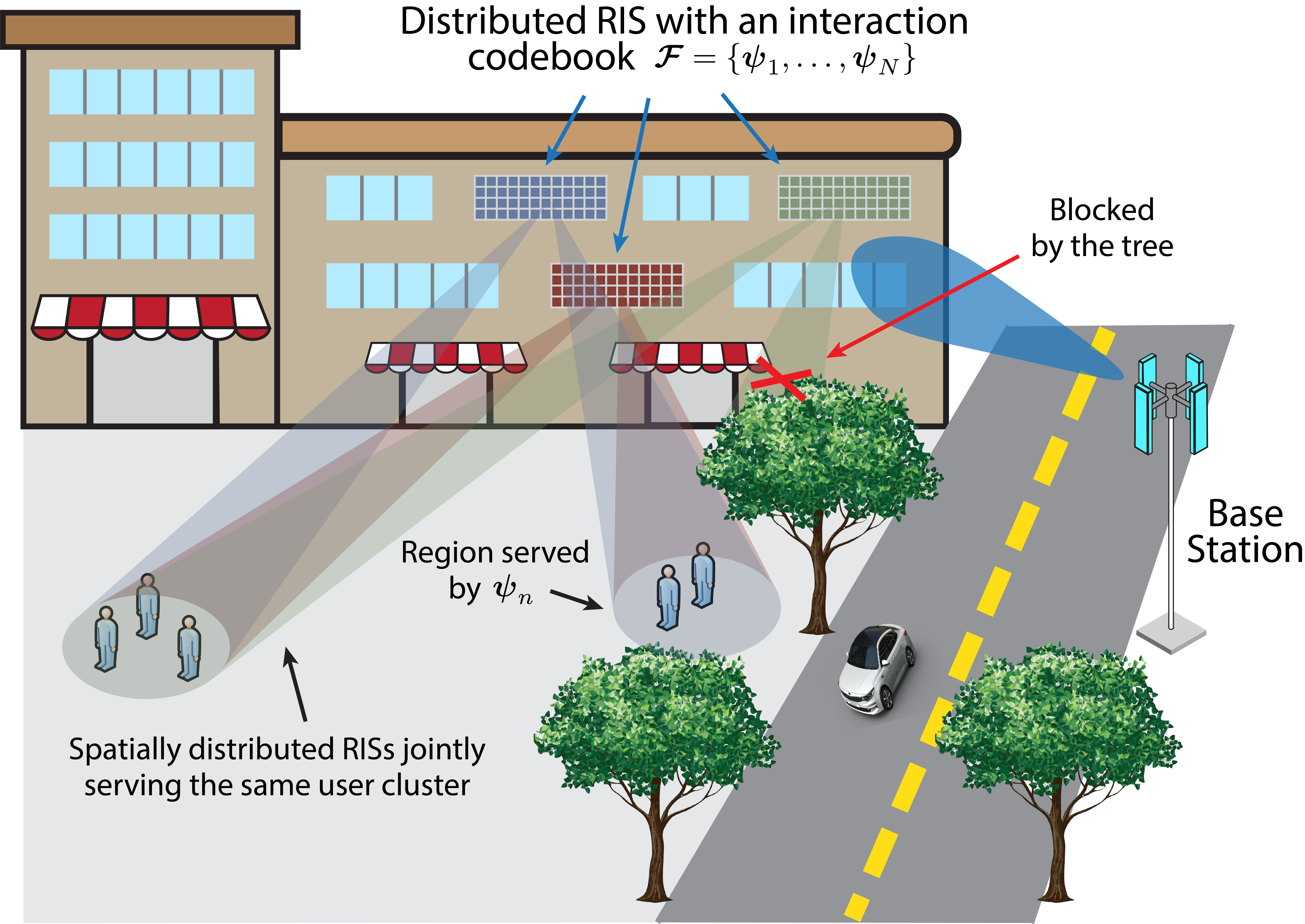
Learning Reflection Codebooks for Reconfigurable Intelligent Surfaces
We develop a novel deep reinforcement learning based framework to efficiently construct the RIS reflection codebook. This framework is devised with a multi-level design process to significantly reduce the ultra high computational complexity associated with the extremely large RIS surfaces. Furthermore, the knowledge learned from one subarray is transferred to other subarrays to further speed up the learning convergence. The proposed framework is able to learn optimized RIS reflection beamforming codebooks that adapt to non-stationary channels and arbitrary RIS geometries, without the knowledge of the channels … read more
Situation-Aware Channel Covariance Prediction for Massive MIMO Systems
We propose a novel deep learning based situation-aware channel covariance prediction solution for Highly-Mobile FDD massive MIMO systems. The BS acquires the locations with different levels of uncertainty, which are in turn leveraged to predict the channel statistics for downlink beamforming design … read more
Learning Beam Codebooks for mmWave and THz Systems
We develop a deep reinforcement learning framework that learns how to optimize the codebook beams relying only on the power measurements. The developed model learns how to adapt the beam patterns based on the surrounding environment, user distribution, hardware impairments, and array geometry, with a full respect of the given constraints … read more
Neural Networks Based mmWave Massive MIMO Beam Codebooks
We develop an efficient online machine learning framework that intelligently learns site and hardware specific codebook beam patterns. This is done by designing a novel complex-valued neural network architecture in which the neuron weights directly model the beamforming weights of the analog phase shifters … read more
mmWave Beam and Blockage Prediction Using Sub-6GHz Channels
We prove and show that under certain conditions, there exists mapping functions that can predict the optimal mmWave beam and blockage status directly from the sub-6 GHz channel. This can be achieved by leveraging a large enough neural network, which is empirically validated by the simulation results….. read more
Deep Reinforcement Learning for Intelligent Reflecting Surfaces
We propose a novel deep reinforcement learning framework for predicting the IRS reflection matrices with minimal beam training overhead. Simulation results show that the proposed online learning framework can converge to the optimal rate that assumes perfect channel knowledge … read more
Deep Learning for Large Intelligent Surfaces
We devise a novel LIS architecture based on sparse channel sensors, where all the LIS elements are passive except for a few elements that are active (connected to the baseband of the LIS controller). We also develop two solutions, based on compressive sensing and deep learning, that design the LIS reflection matrices with negligible training overhead … read more
Channel Estimation for Massive MIMO with One-Bit ADCs
We propose a deep learning based framework for the channel estimation problem in massive MIMO systems with 1-bit ADCs, where the prior channel estimation observations and deep neural networks are exploited to learn the mapping from the received highly quantized measurements to the channels. Furthermore, we derive the structure of the pilot sequence under which the mapping from the quantized measurements to the full-resolution channel vector is bijective, which leads to an interesting and counter-intuitive observation that when more antennas are employed at the base station, fewer pilots are required in order to achieve the same level of channel estimation performance … read more
Mapping Channels in Space and Frequency
Can we map the channels at one set of antennas and one frequency band to the channels at another set of antennas- possibly at a different location and a different frequency band? If this channel-to-channel mapping is possible, we can expect dramatic gains for massive MIMO systems. In this work, we introduce the new concept of channel mapping in space and frequency, where the channels at one set of antennas and one frequency band are mapped to the channels at another set of antennas and frequency band. First, we prove that this channel-to-channel mapping function exists under certain conditions. Then, we leverage the powerful learning capabilities of deep neural networks to efficiently learn this non-trivial channel mapping function, which is also confirmed by the simulation results … read more
Direct Hybrid Precoding in mmWave Massive MIMO Systems
We propose a novel neural network architecture, that we call an auto-precoder, and a deep-learning based approach that jointly senses the mmWave channel and designs the hybrid precoding matrices with only a few training pilots. The proposed approach significantly reduces the training overhead compared to classical (non-machine learning) solutions … read more
Channel Covariance Prediction using Generative Adversarial Networks
We develop a novel solution that exploits machine learning tools, namely conditional generative adversarial networks (GAN), to learn the functions between the environment and the channel covariance matrices, which dramatically reduces the training overhead needed to obtain the channel knowledge … read more
Millimeter Wave Beam Prediction Based on Multipath Signature
We develop a novel integrated machine learning and coordinated beamforming solution to overcome the challenges encountered in the highly-mobile mmWave systems, such as coverage, reliability and latency, etc., which has the potential of supporting high mobility applications in the real-world mmWave systems … read more
Blockage Prediction and Proactive Handoff
We propose a novel machine learning based solution to tackle the reliability and latency challenges in mmWave MIMO systems. Simulation results show that the developed deep learning based strategy successfully predicts blockage/hand-off in close to 95% of the times … read more
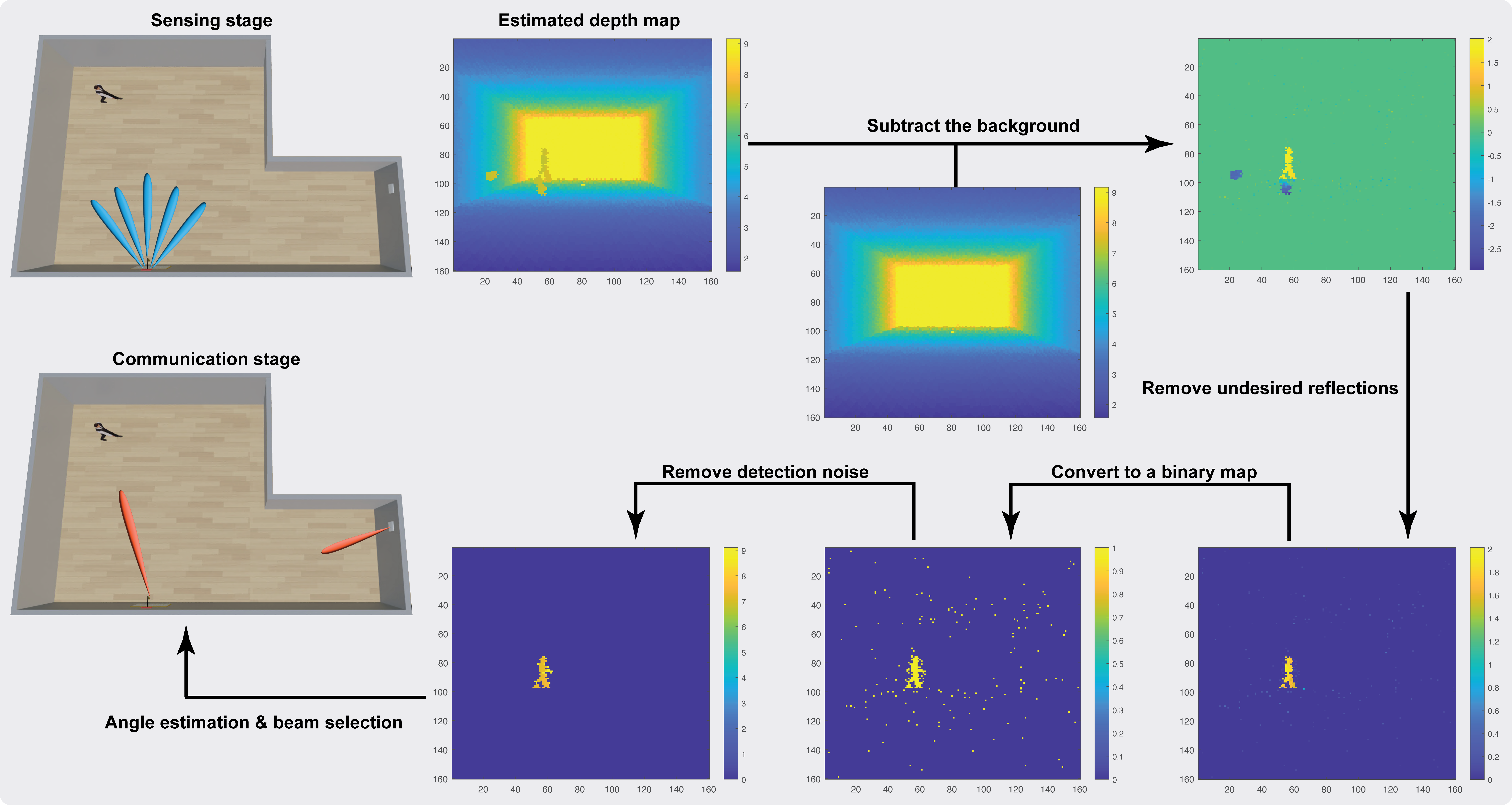
Integrated Imaging and Communication with Reconfigurable Intelligent Surfaces
Reconfigurable intelligent surfaces, with their large number of antennas, offer an interesting opportunity for high spatial-resolution imaging. In this paper, we propose a novel RIS-aided integrated imaging and communication system that can reduce the RIS beam training overhead for communication by leveraging the imaging of the surrounding environment. In particular, using the RIS as a wireless imaging device, our system constructs the scene depth map of the environment, including the mobile user. Then, we … read more
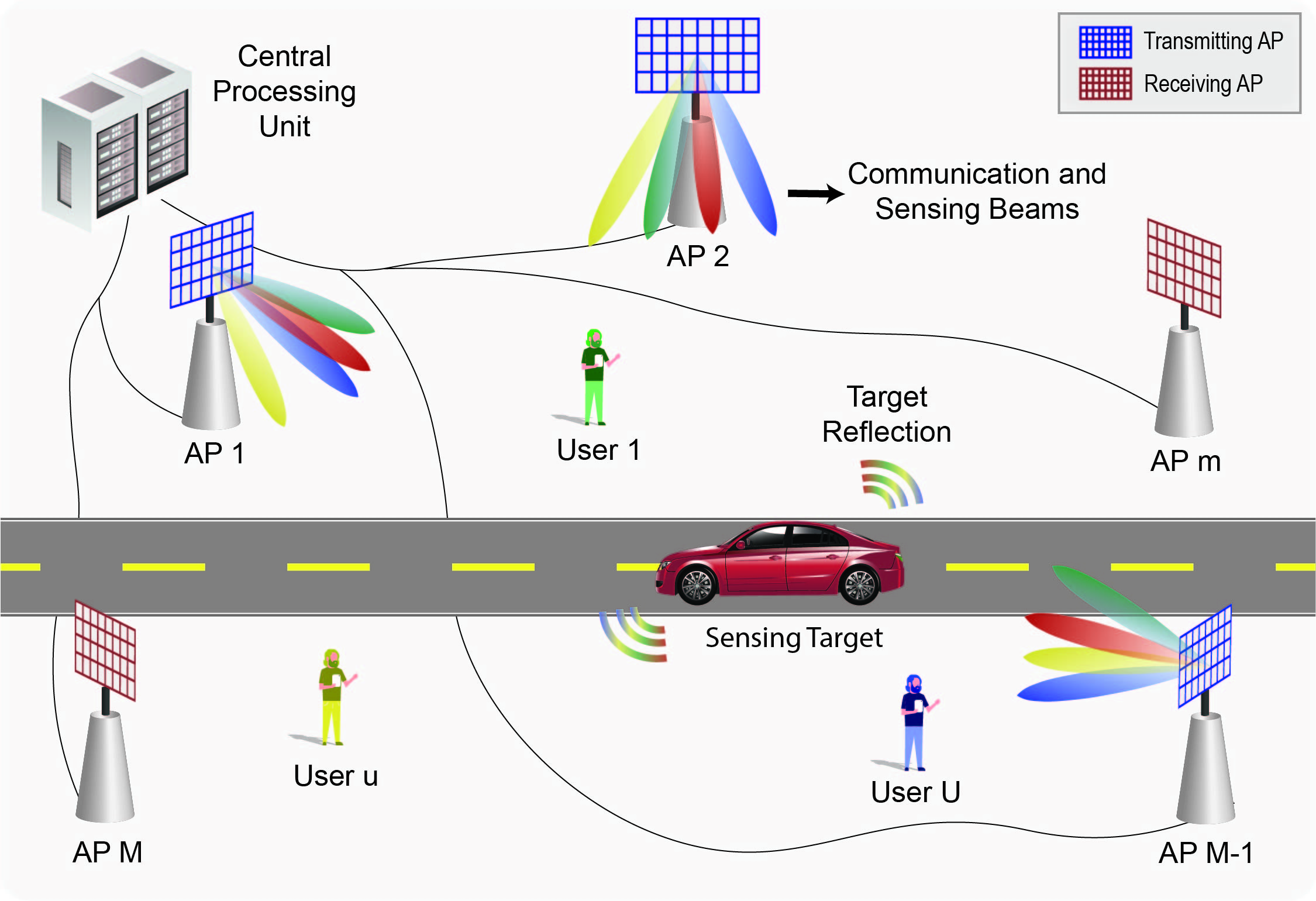
Cell-free ISAC MIMO Systems: Joint Sensing and Communication Beamforming
This paper considers a cell-free integrated sensing and communication (ISAC) MIMO system, where distributed MIMO access points are jointly serving the communication users and sensing the targets. For this setup, we first develop two baseline approaches that separately design the sensing and communication beamforming vectors. Then, we consider the joint sensing and communication (JSC) beamforming design and derive the optimal structure of these JSC beamforming vectors based on a max-min fairness formulation. The results … read more
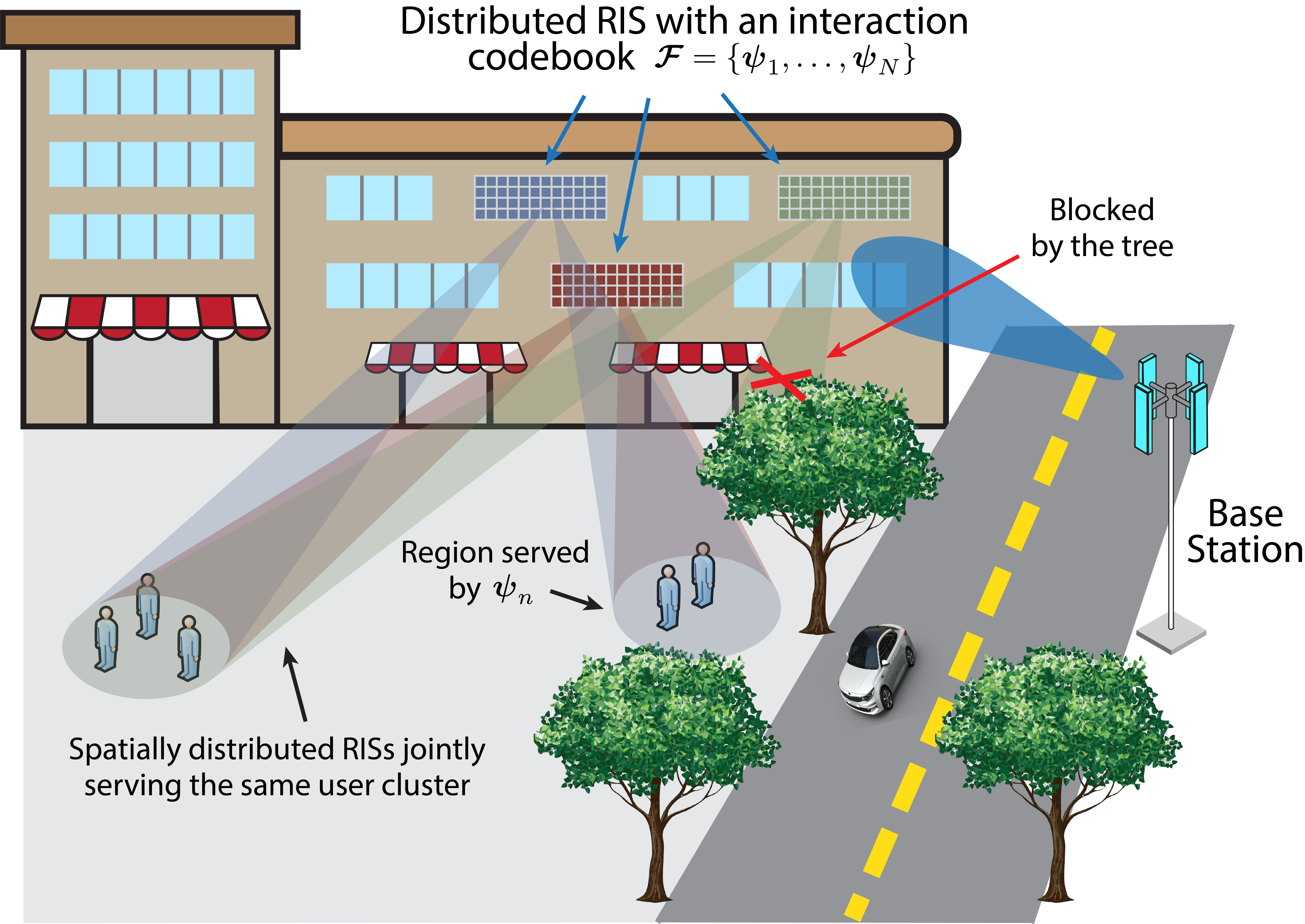
Learning Reflection Codebooks for Reconfigurable Intelligent Surfaces
We develop a novel deep reinforcement learning based framework to efficiently construct the RIS reflection codebook. This framework is devised with a multi-level design process to significantly reduce the ultra high computational complexity associated with the extremely large RIS surfaces. Furthermore, the knowledge learned from one subarray is transferred to other subarrays to further speed up the learning convergence. The proposed framework is able to learn optimized RIS reflection beamforming codebooks that adapt to non-stationary channels and arbitrary RIS geometries, without the knowledge of the channels … read more
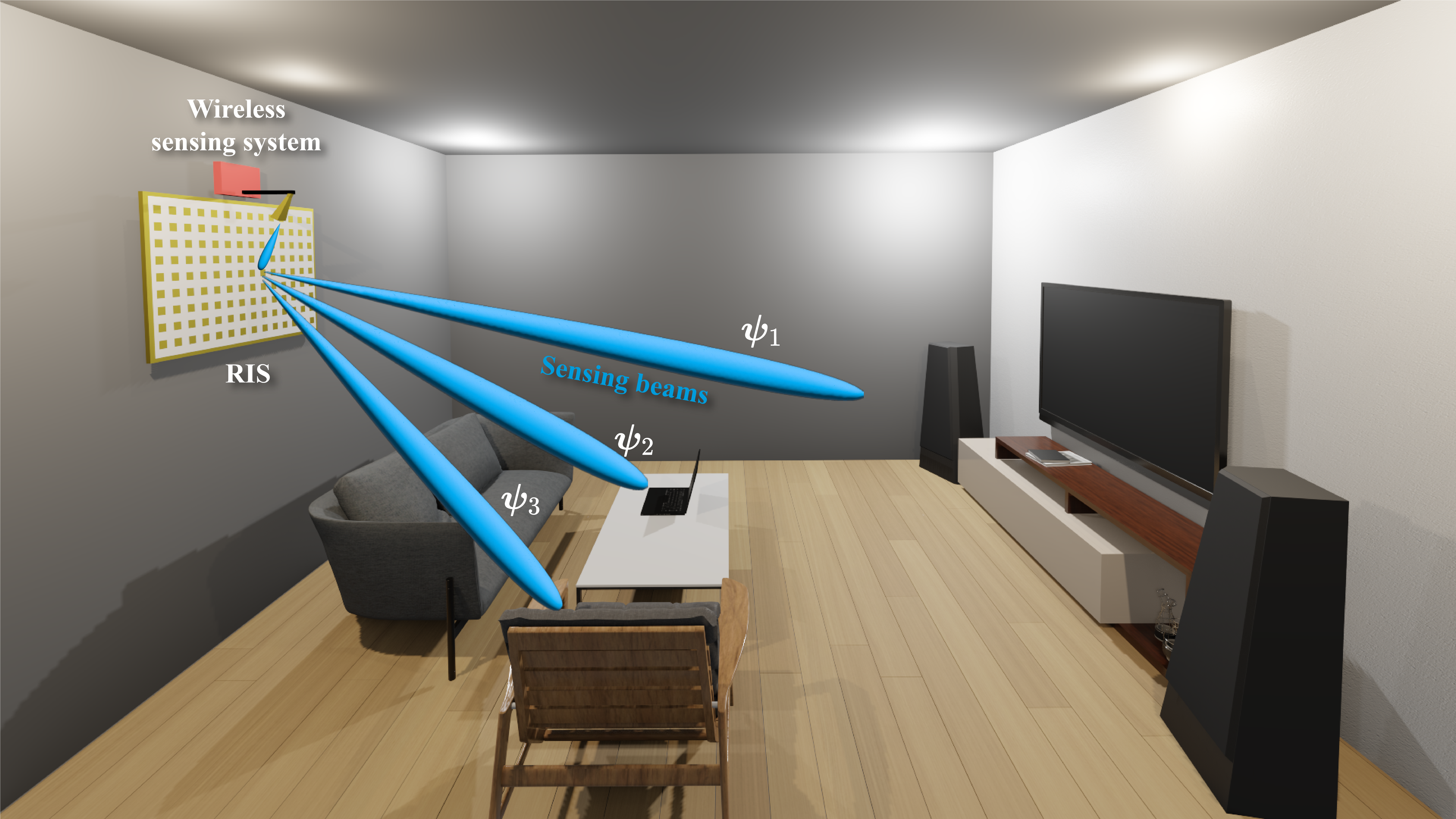
Reconfigurable Intelligent Surface Aided Wireless Sensing for Scene Depth Estimation
We propose to employ RIS-aided wireless sensing systems for scene depth estimation. We develop a comprehensive framework for building accurate depth maps using RIS-aided mmWave sensing systems. In this framework, we propose a new RIS interaction codebook capable of creating a sensing grid of reflected beams that meets the desirable characteristics of efficient scene depth map construction … read more
Enabling Cell-Free Massive MIMO Systems with Wireless mmWave Fronthaul
We propose two architectures for cell-free massive MIMO systems based on wireless fronthaul that is operating at a higher-band compared to the access links: (i) A wireless-only fronthaul architecture where the CPU has a wireless fronthaul link to each AP, and (ii) a mixed-fronthaul architecture where the CPU has a wireless link to each cluster of APs that are connected together via optical fibers … read more
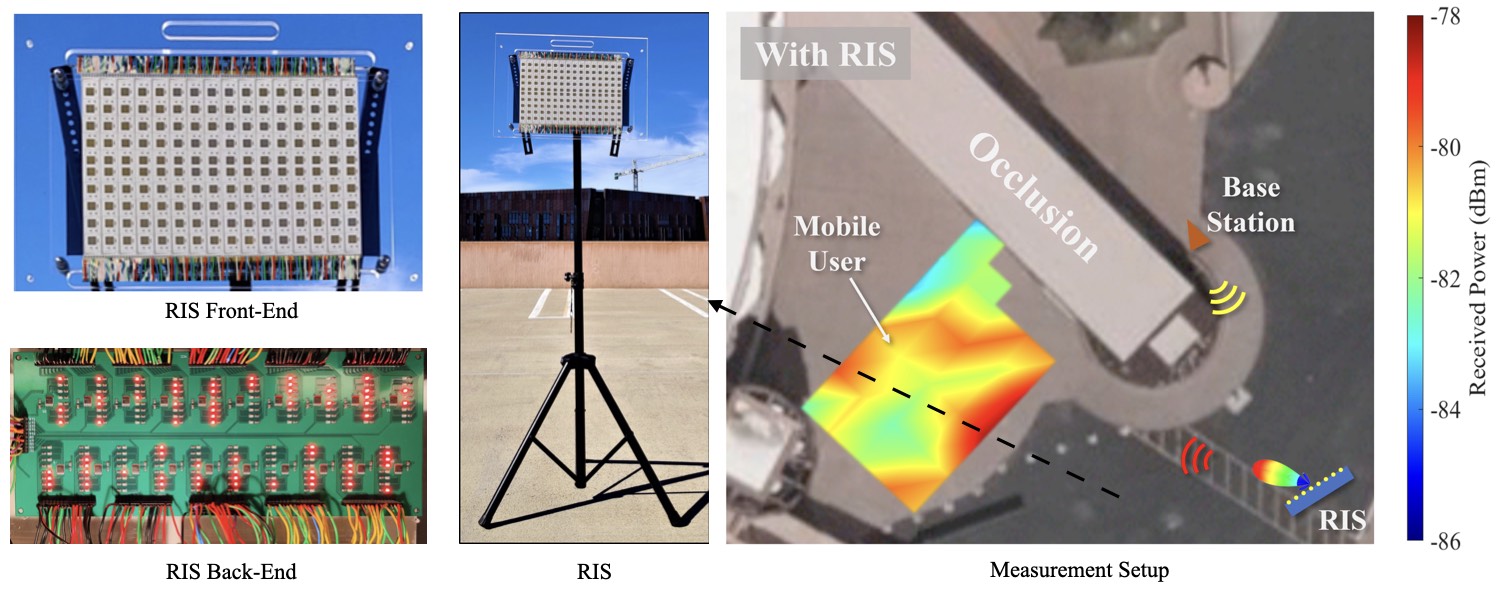
Reconfigurable Intelligent Surfaces in Real-World Environment
Reconfigurable intelligent surfaces (RISs) have promising coverage and data rate gains for wireless communication systems in 5G and beyond. To draw accurate insights about the actual performance of these systems, this paper develops an RIS proof-of-concept prototype and extensively evaluates its potential gains in the field and under realistic wireless communication settings … read more
Deep Reinforcement Learning for Intelligent Reflecting Surfaces
We propose a novel deep reinforcement learning framework for predicting the IRS reflection matrices with minimal beam training overhead. Simulation results show that the proposed online learning framework can converge to the optimal rate that assumes perfect channel knowledge … read more
Deep Learning for Large Intelligent Surfaces
We devise a novel LIS architecture based on sparse channel sensors, where all the LIS elements are passive except for a few elements that are active (connected to the baseband of the LIS controller). We also develop two solutions, based on compressive sensing and deep learning, that design the LIS reflection matrices with negligible training overhead … read more
Relay Aided Intelligent Reconfigurable Surfaces
This paper proposes a novel relay-aided intelligent reconfigurable surface (IRS) architecture for future wireless communication systems. The proposed architecture, which consists of two side-by-side intelligent surfaces connected via a full-duplex relay, has the potential of achieving the promising IRS gains while requiring much less numbers of antenna elements … read more
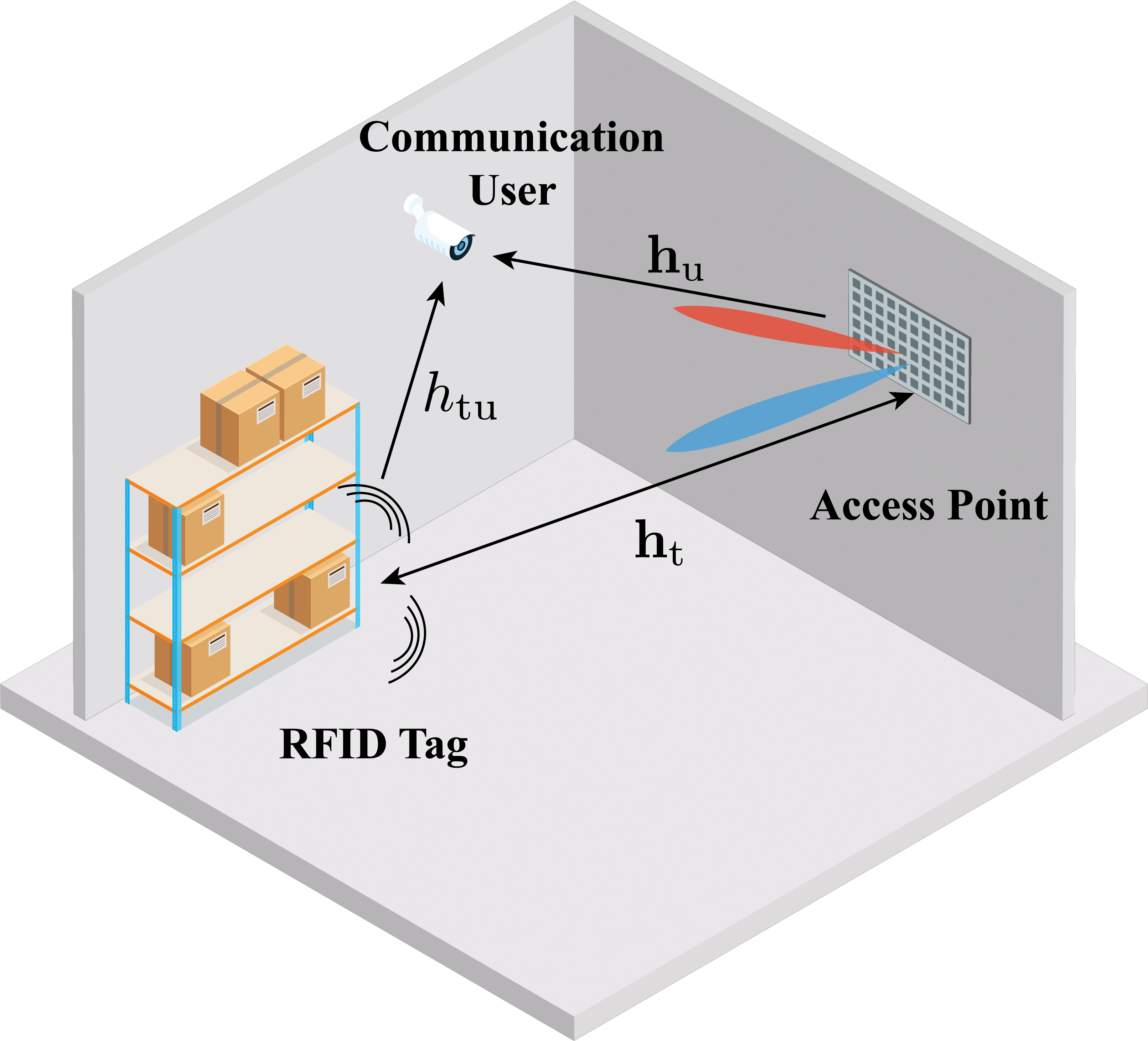
ISAC with Backscattering RFID Tags: Joint Beamforming Design
In this paper, we explore an integrated sensing and communication (ISAC) system with backscattering RFID tags. In this setup, an access point employs a communication beam to serve a user while leveraging a sensing beam to detect an RFID tag. Under the total transmit power constraint of the system, our objective is to design sensing and communication beams by considering the tag detection and communication requirements. First, we adopt zero-forcing to design the beamforming vectors, followed by solving a convex optimization problem to determine the power allocation between sensing and communication. Then, we study a joint beamforming design problem with the goal of minimizing the total transmit power while satisfying the tag detection and communication requirements. To resolve this, we re-formulate the non-convex constraints into convex second-order cone constraints. … read more
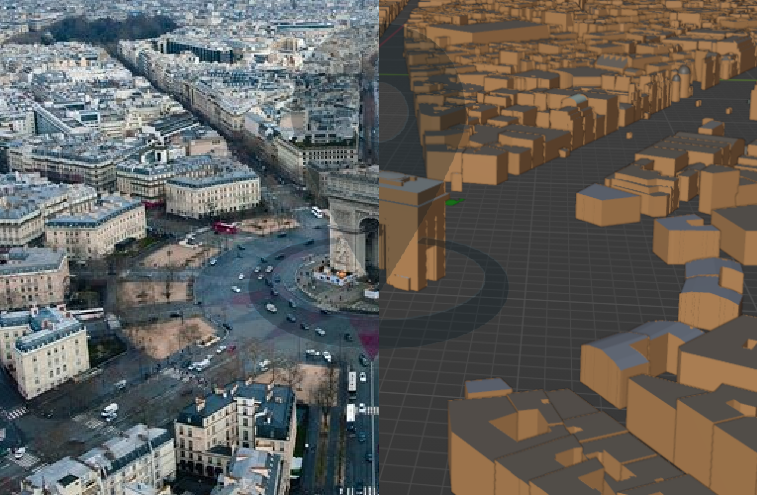
Localization in Digital Twin MIMO Networks: A Case for Massive Fingerprinting
This work proposes using Digital Twins to significantly reducing human effort in building fingerprinting databases. This involves ray-tracing on a digital replica of the environment across several frequency bands and beamforming configurations. Our experiments show sub-meter localization errors on a NLoS location 95% of the time using sensible user measurement report sizes. Results highlight the promising potential of the proposed digital twin approach for ubiquitous wide-area 6G localization. (read more)
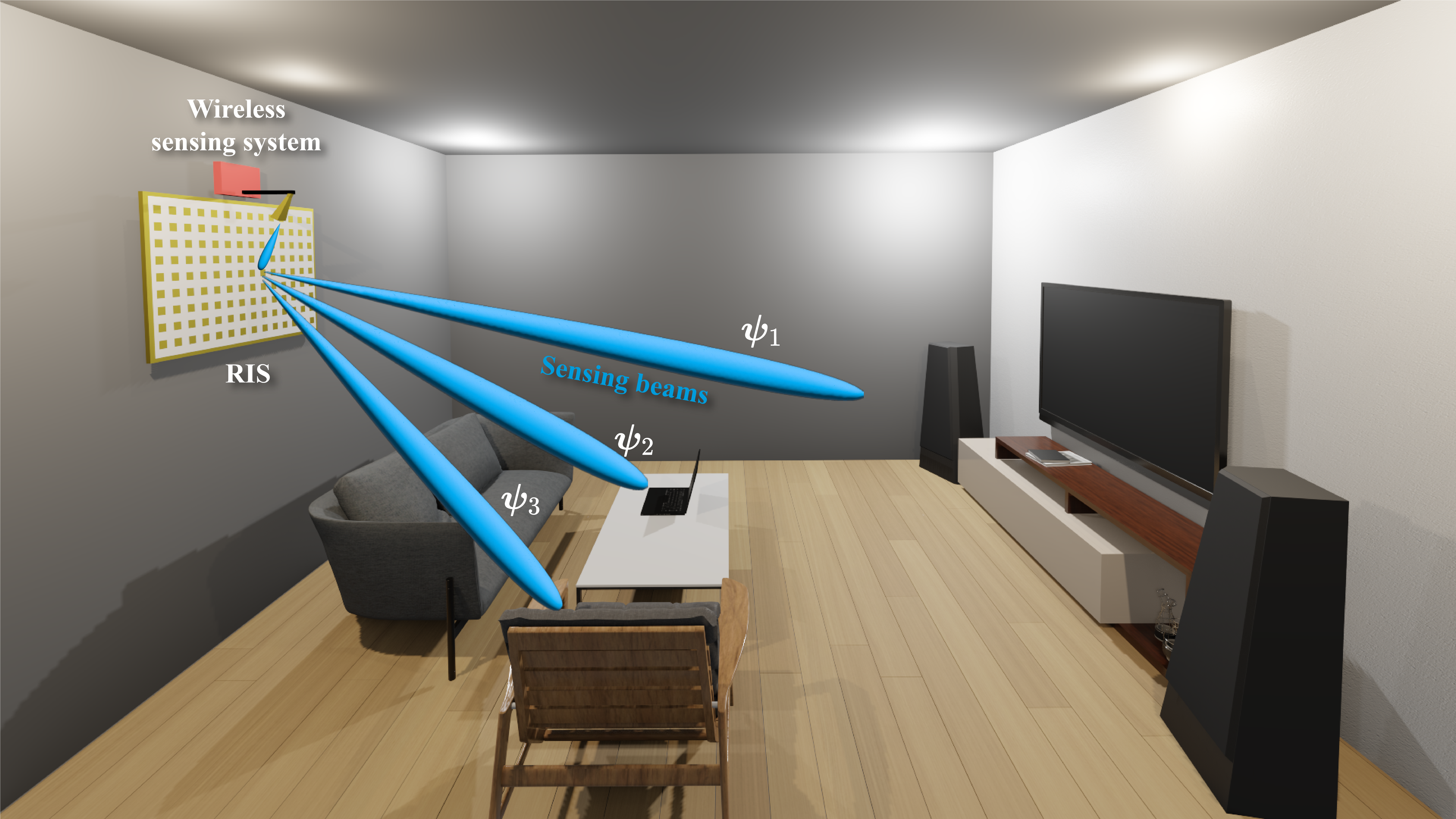
Reconfigurable Intelligent Surface Aided Wireless Sensing for Scene Depth Estimation
We propose to employ RIS-aided wireless sensing systems for scene depth estimation. We develop a comprehensive framework for building accurate depth maps using RIS-aided mmWave sensing systems. In this framework, we propose a new RIS interaction codebook capable of creating a sensing grid of reflected beams that meets the desirable characteristics of efficient scene depth map construction … read more
mmWave MIMO Based Depth Maps for Wireless AR/VR
We propose a wireless AR/VR depth map construction framework using mmWave communication transceivers. This sensing framework is used for building depth maps under the constraints imposed by mmWave communication hardware and frame structure. We develop a new sensing beamforming codebook specific for depth map construction objective … read more
Blockage Prediction Using Wireless Signatures in a Real-World Demonstration
The blockage prediction approaches in this paper are based on the following simple observation: Consider a fixed TX and RX in a certain environment with a LOS path. If an object moves in this environment till it blocks this LOS path, then, during the movement, the object acts like a scatterer for the signal propagating from the TX to the RX. The received signal during this interval will experience a constructive and destructive interference from the LOS ray and the ray scattered on the moving object. … read more
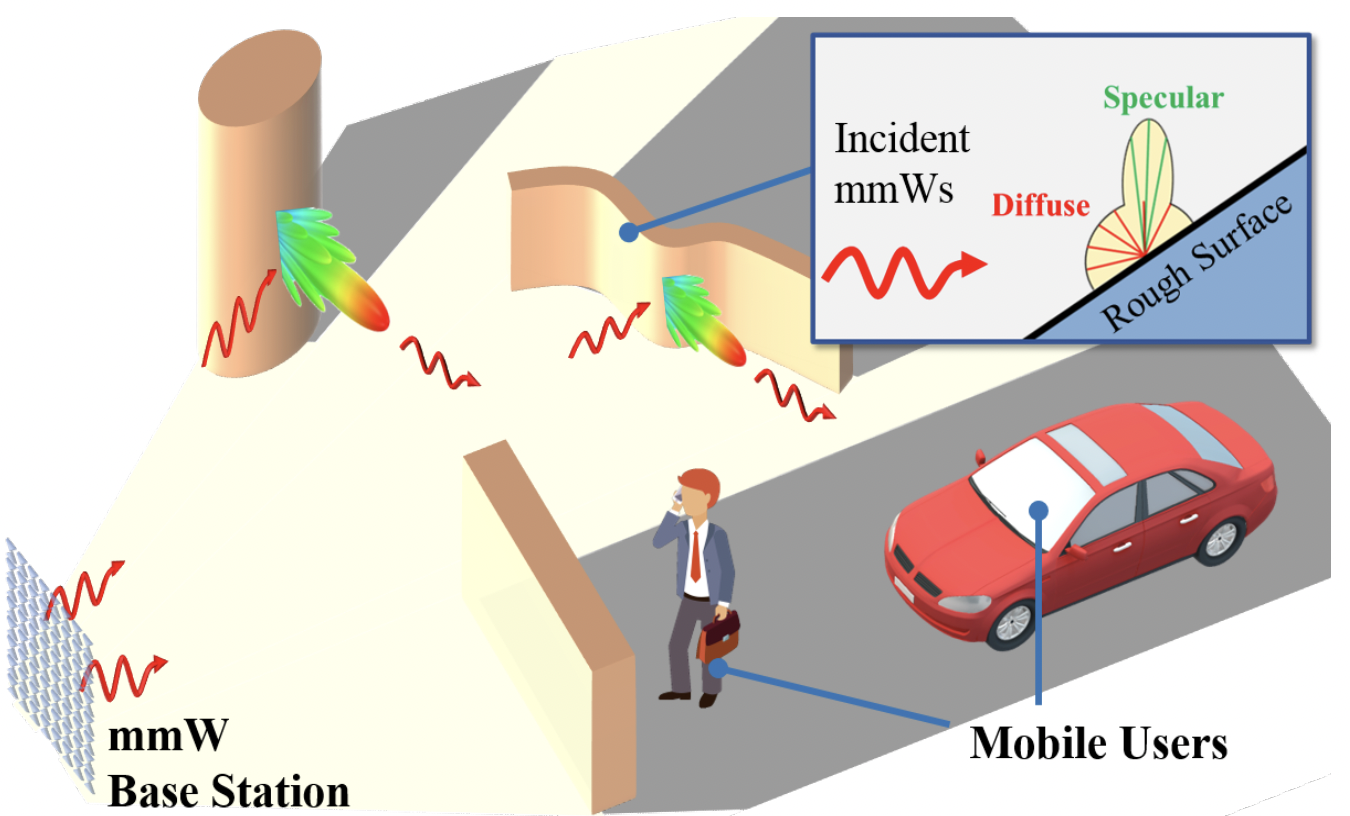
mmWave Imaging and Communications for Simultaneous Localization and Mapping
In this work, we propose a novel approach for high accuracy user localization by merging tools from both millimeter wave (mmWave) imaging and communications. The key idea of the proposed solution is to leverage mmWave imaging to construct a high-resolution 3D image of the line-of-sight (LOS) and non-line-of-sight (NLOS) objects in the environment at one antenna array. Then, uplink pilot signaling with the user is used to estimate the angle-of-arrival and time-of-arrival of the dominant channel paths. By projecting the AoA and ToA information on the 3D mmWave images of the environment, the proposed solution can locate the user with a sub-centimeter accuracy … read more
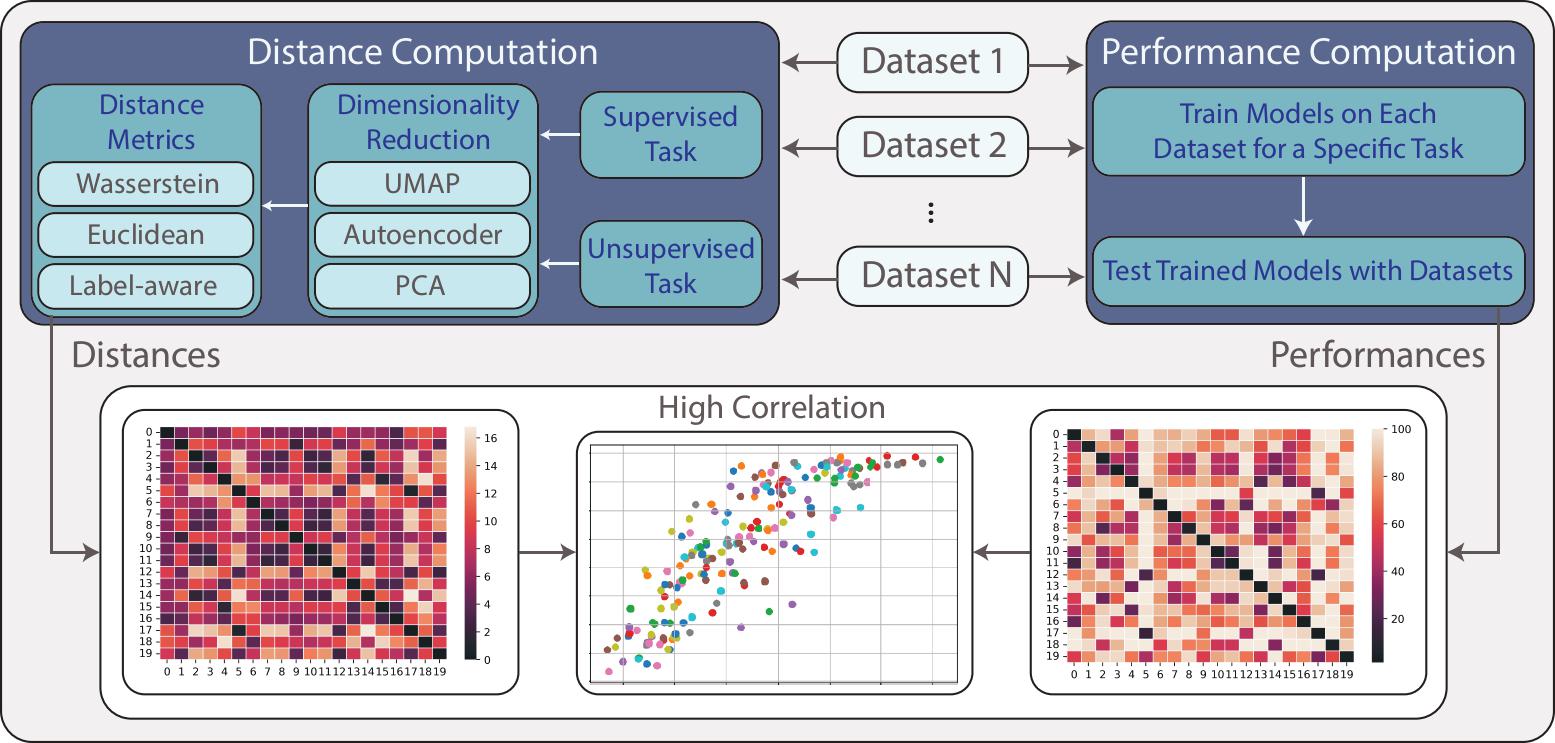
A Dataset Similarity Evaluation Framework for Wireless Communications and Sensing
Given the critical discrepancies between synthetic and real-world datasets, there is a pressing need to quantify these differences and how they affect model performance to ensure effective generalization and reliable performance in real world deployments. This paper proposes a task-specific, model-agnostic metric for measuring ‘dataset distance’ and effectively assess and compare the realism and quality of datasets. Such a metric would enable real-world data augmentation, facilitate effective benchmarking, and help in retraining decisions. … read more
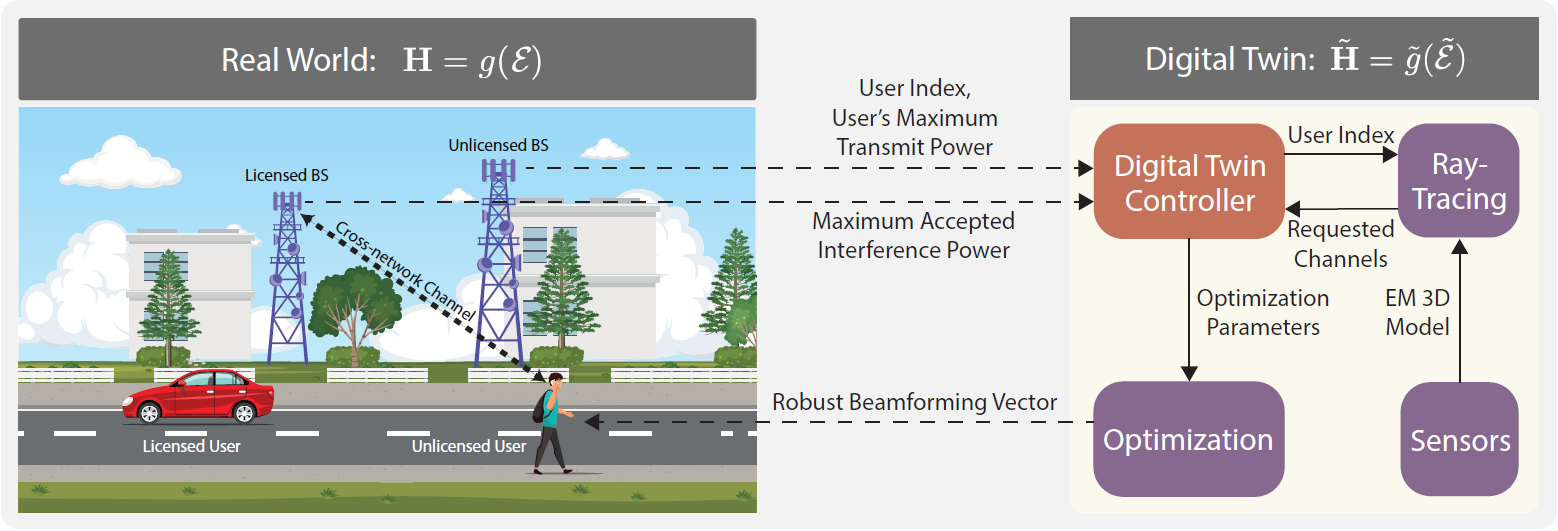
Digital Twin for Spectrum Sharing and Coexistence: Coordinating the Uncoordinated
Spectrum sharing provides a solution to the limited spectrum available in high-demand wireless networks, but ensuring compliance with interference regulations in licensed networks is particularly challenging for uncoordinated systems. In this paper, we introduce a novel approach using digital twins (DTs) to coordinate networks and approximate wireless channels, maximizing transmission in unlicensed networks. To address potential DT channel errors, we propose two robust beamforming designs that meet interference constraints over 97.5% of the time, demonstrating the effectiveness of this approach.
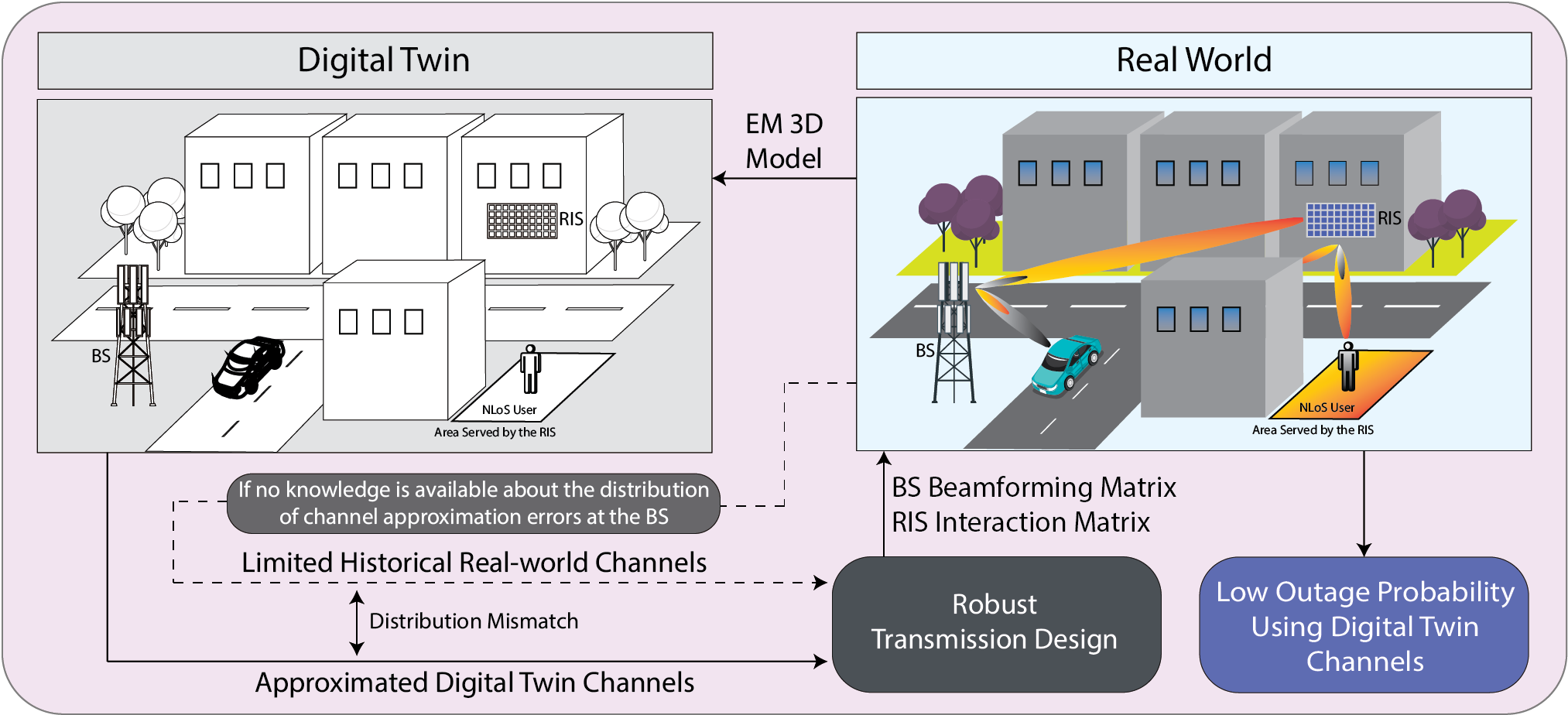
Digital Twin Aided RIS Communication: Robust Beamforming and Interference Management
Reconfigurable intelligent surfaces (RISs) are envisioned to play a key role in future wireless communication networks. However, channel estimation in RIS-aided wireless networks is challenging due to their passive nature and the large number of reflective elements, leading to high channel estimation overhead. Additionally, conventional methods like beam sweeping, which do not rely on explicit channel state information, often struggle in managing interference in multi-user networks. In this paper, we … (read more)
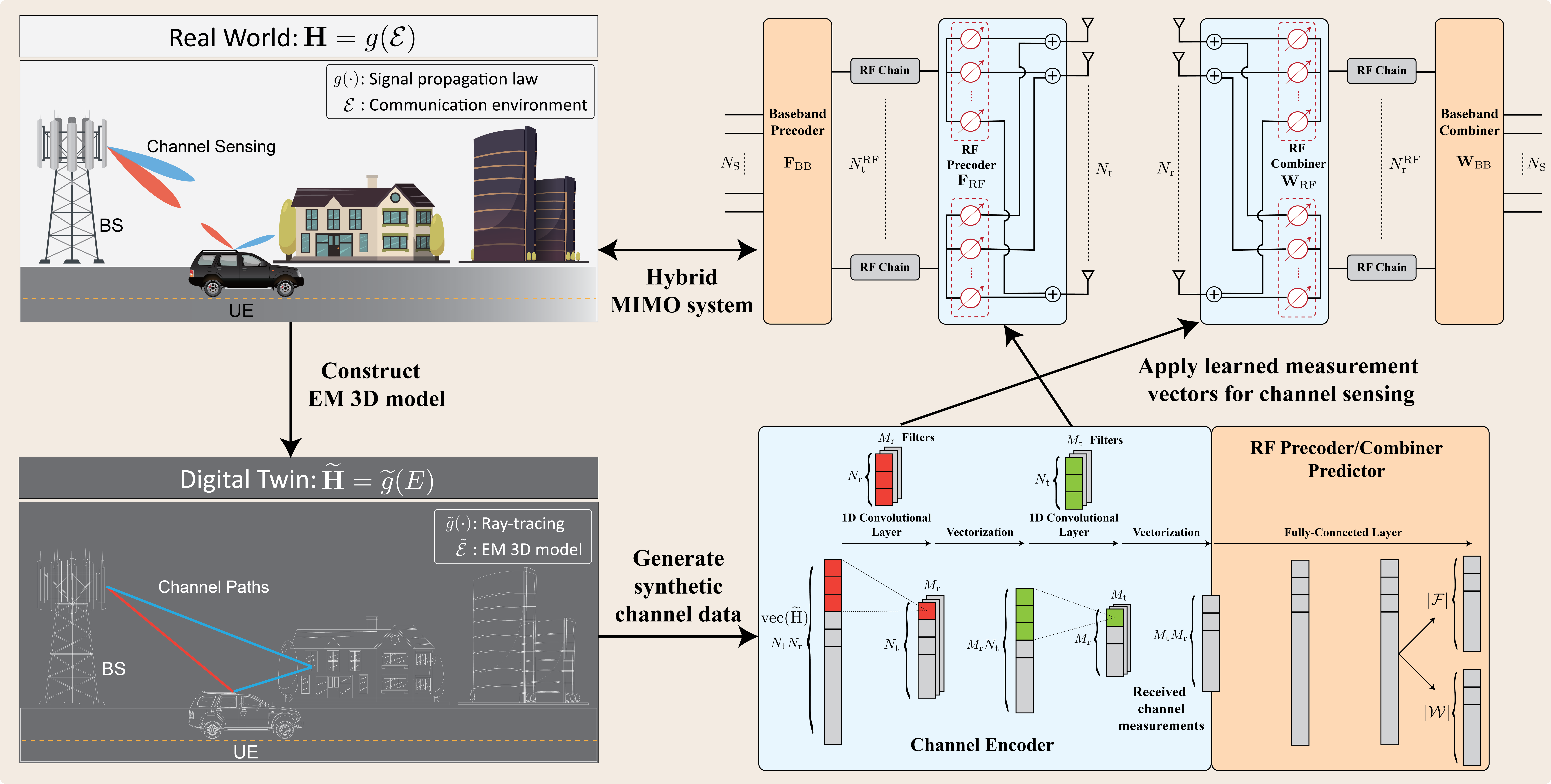
Digital Twin Aided Compressive Sensing: Enabling Site-Specific MIMO Hybrid Precoding
Compressive sensing is a promising solution for the channel estimation in multiple-input multiple-output (MIMO) systems with large antenna arrays and constrained hardware. Utilizing site-specific channel data from real-world systems, deep learning can be employed to learn the compressive sensing measurement vectors with minimum redundancy, thereby focusing sensing power on promising spatial directions of the channel. Collecting real-world channel data, however, is challenging due to the high overhead resulting from the large number of antennas and hardware constraints. In this paper, we … (read more)
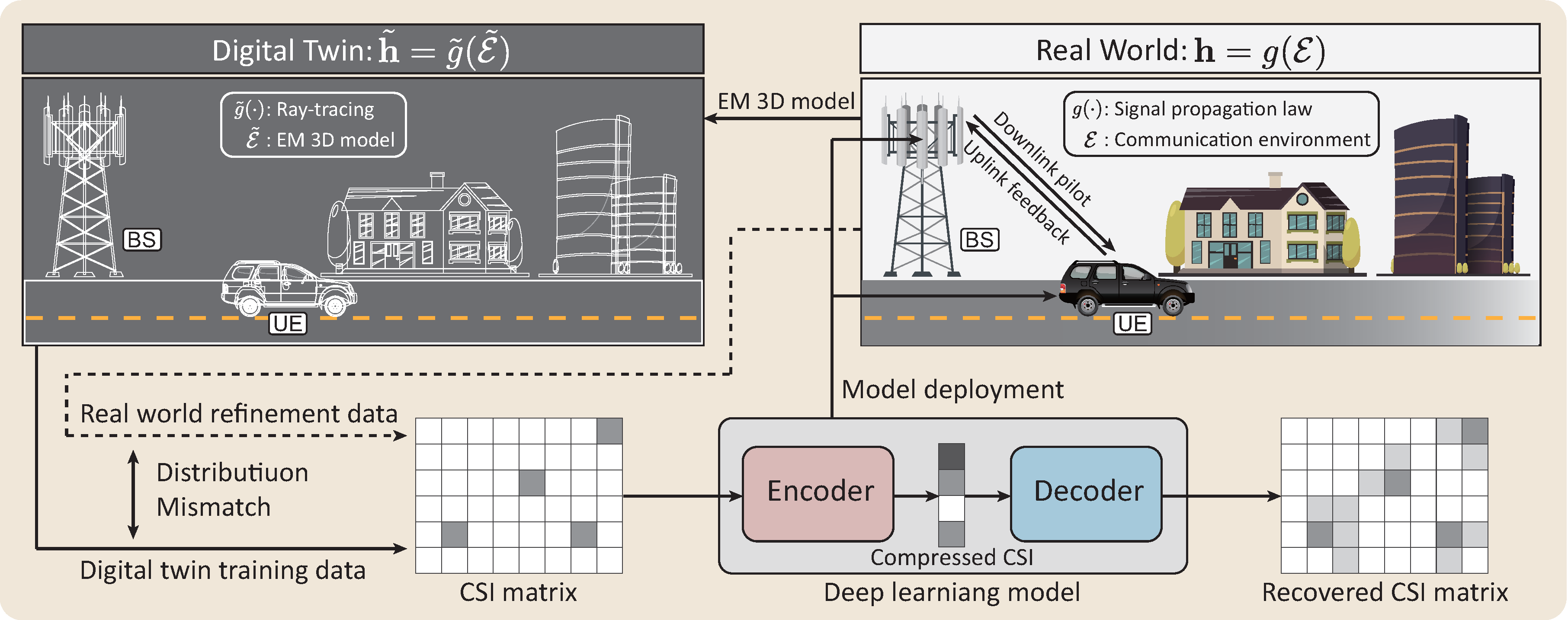
Digital Twin Aided Massive MIMO: CSI Compression and Feedback
Deep learning (DL) approaches have demonstrated high performance in the CSI compression task. However, the DL approaches requires extensive training data. Thee significant data collection overhead hinders the DL approaches from scaling to a large number of sites. We propose a novel direction that utilizes site-specific digital twins to aid the training of DL models. The proposed digital twin approach generates site-specific synthetic CSI data from the EM 3D model and ray tracing, which can then be used to train the DL model without real-world data collection.
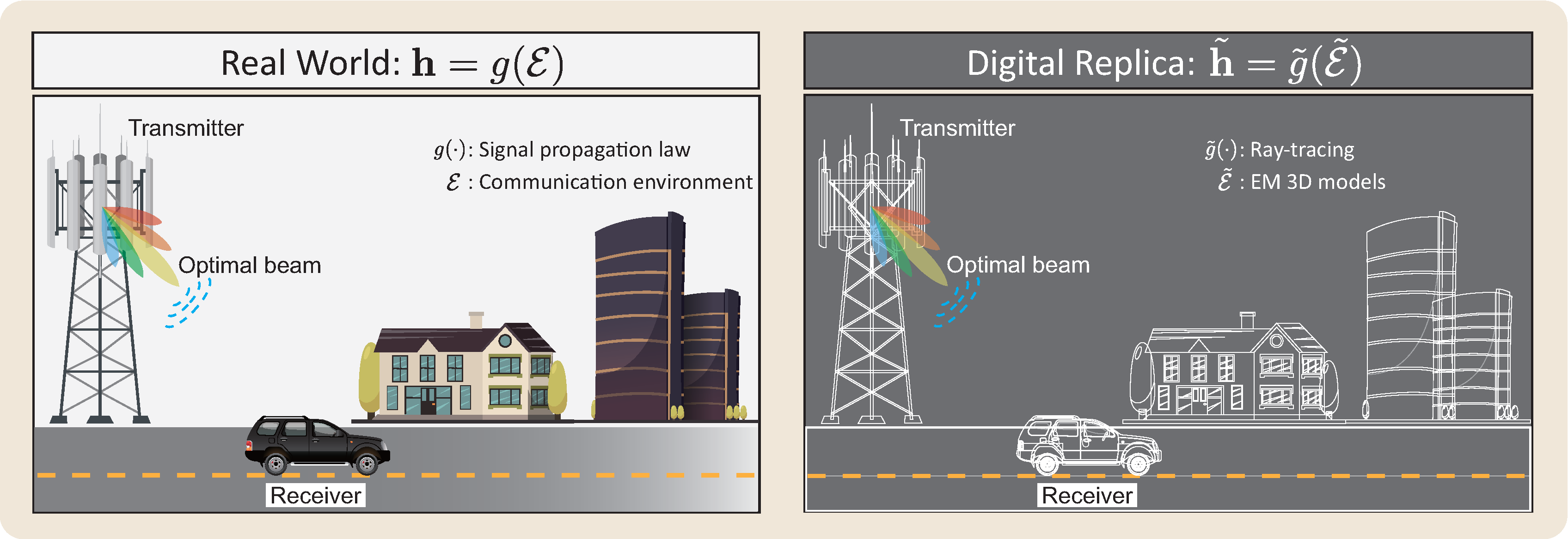
Digital Twin Based Beam Prediction: Can we Train in the Digital World and Deploy in Reality?
Realizing the potential gains of large-scale MIMO requires accurate estimation of their channels. This, however, is typically associated with high channel acquisition overhead. Machine and deep learning represent promising approaches to overcome these challenges thanks to their powerful ability to learn from prior observations and side information. Training machine and deep learning models, however, leads to large data collection overhead. To address this challenge, we propose a novel direction that utilizes digital replicas of the physical world to reduce or even eliminate the MIMO channel acquisition overhead… (read more)
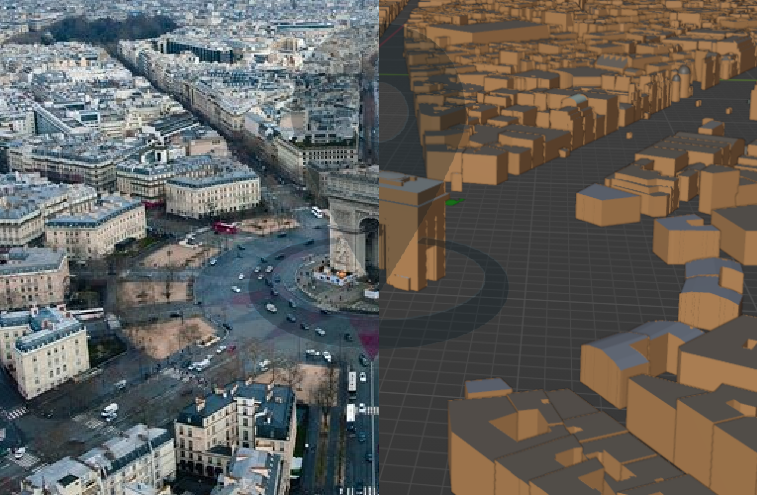
Localization in Digital Twin MIMO Networks: A Case for Massive Fingerprinting
This work proposes using Digital Twins to significantly reducing human effort in building fingerprinting databases. This involves ray-tracing on a digital replica of the environment across several frequency bands and beamforming configurations. Our experiments show sub-meter localization errors on a NLoS location 95% of the time using sensible user measurement report sizes. Results highlight the promising potential of the proposed digital twin approach for ubiquitous wide-area 6G localization. (read more)
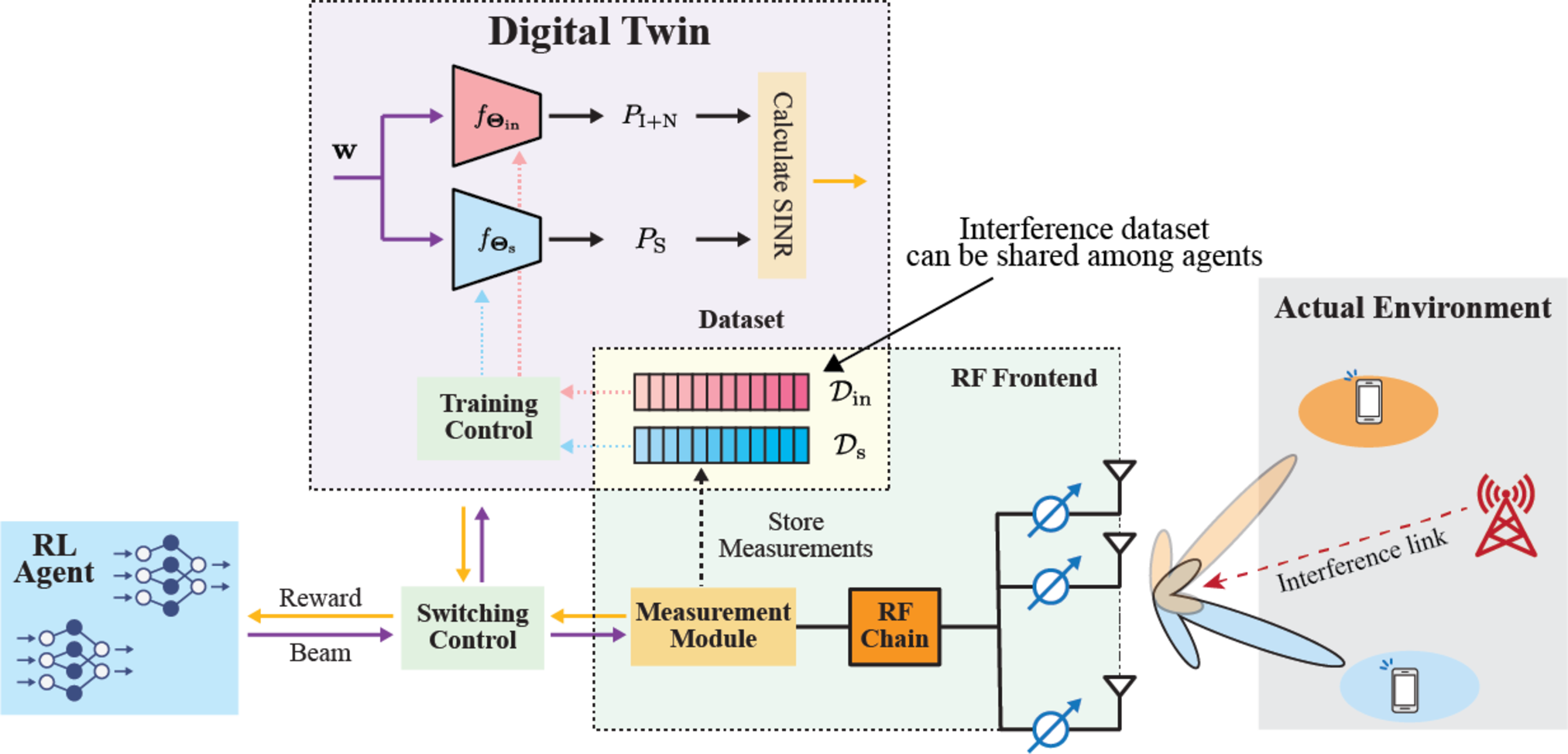
A Digital Twin Assisted Framework for Interference Nulling in Millimeter Wave MIMO Systems
Millimeter wave (mmWave) and terahertz MIMO systems rely on pre-defined beamforming codebooks for both initial access and data transmission. However, most of the existing codebooks adopt pre-defined beams that focus mainly on improving the gain of their target users, without taking interference into account, which could incur critical performance degradation in dense networks. To address this problem, in this paper, we propose …(read more)