A Dataset Similarity Evaluation Framework for
Wireless Communications and Sensing
Asilomar Conference on Signals, Systems, and Computers 2024
João Morais¹, Sadjad Alikhani¹, Akshay Malhotra², Shahab Hamidi-Rad², Ahmed Alkhateeb¹
¹Wireless Intelligence Lab, Arizona State University, USA ²Interdigital Inc., USA
Abstract
In wireless communications and sensing, machine learning models are typically trained on synthetic data that may not accurately reflect real-world conditions, leading to an overestimation of model performance. Given the critical discrepancies between synthetic and real-world datasets, there is a pressing need to quantify these differences and how they affect model performance to ensure effective generalization and reliable performance in real world deployments. This paper proposes a task-specific, model-agnostic metric for measuring ‘dataset distance’ and effectively assess and compare the realism and quality of datasets. Such a metric would enable real-world data augmentation, facilitate effective benchmarking, and help in retraining decisions when altering deployment environments, such as changing sites or adjusting frequencies.
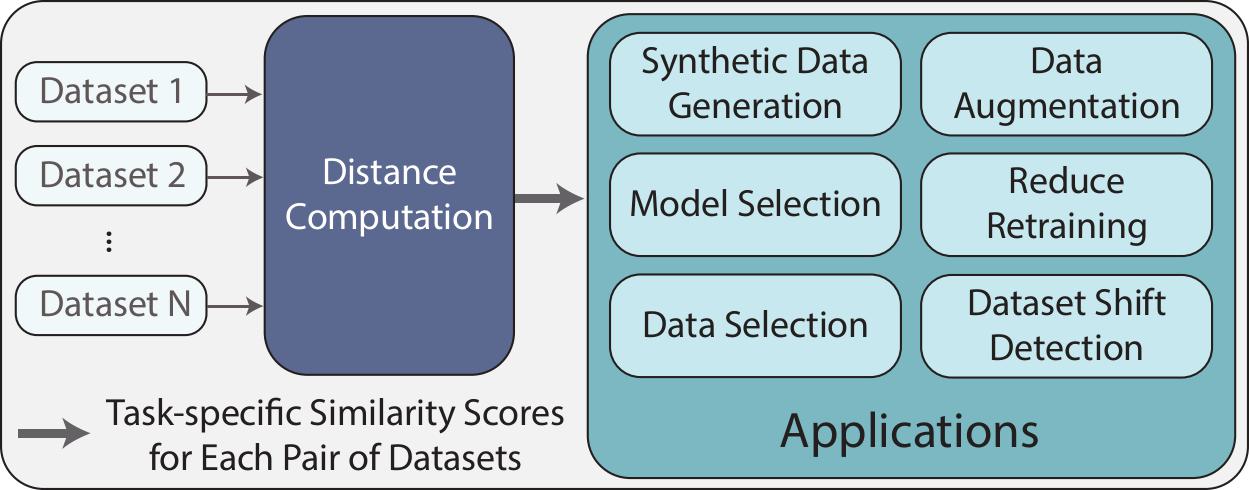
Fig. 1. Applications enabled by dataset distance computation.
Quantifying the difference between two datasets has a wide range of applications, some depicted in the figure above.
Proposed Solution
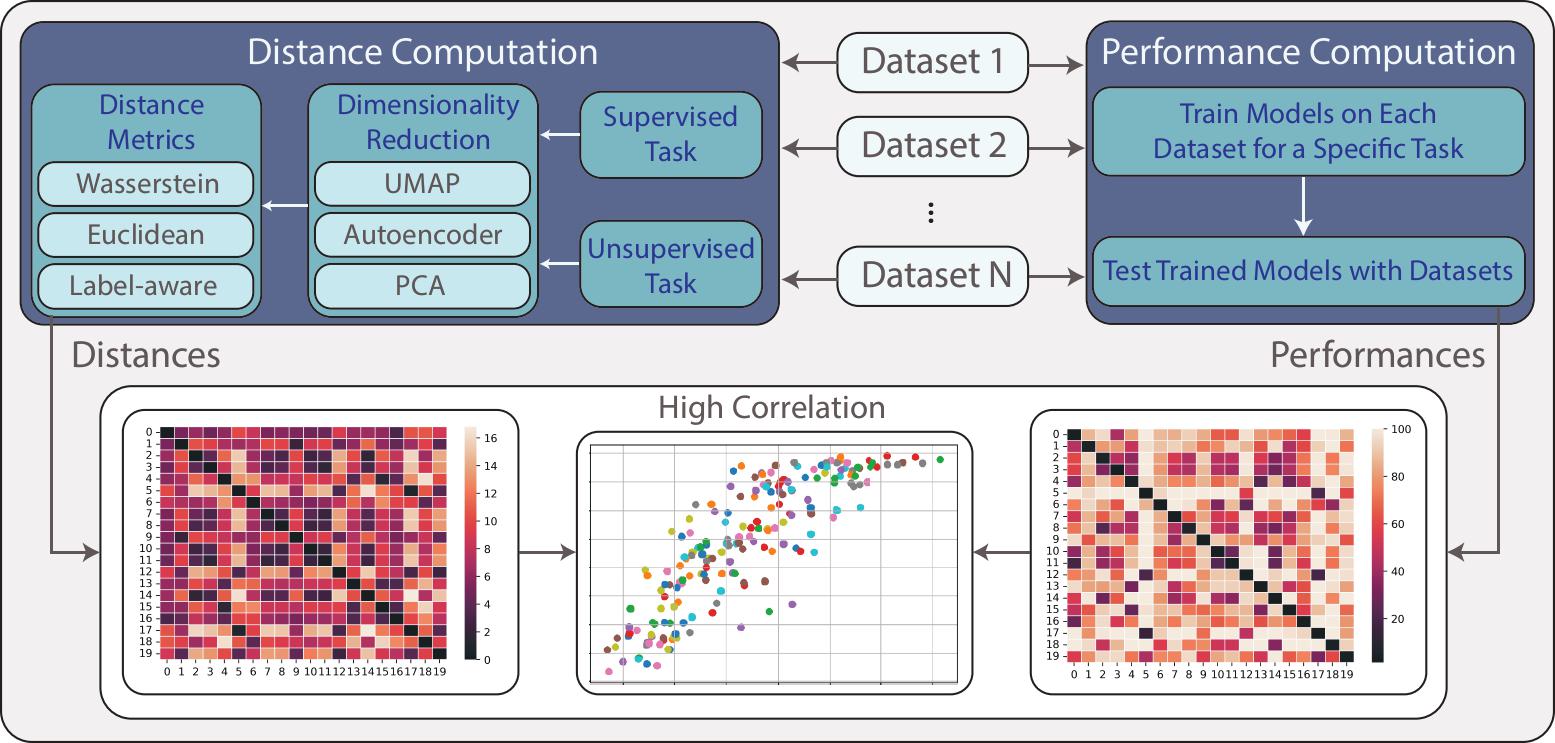
Fig. 2. Framework system for assessing the suitability of a distance function to task, model, and a set of datasets in terms of how such a function outputs distances that correlate with performances in the specific task.
To assess how well several distance methods can predict model performance, we design this framework to correlate such methods with the experienced performance drop from models trained in one dataset and tested on the others. Such framework allowed us to propose two metrics based on UMAP latent spaces that outperform other distance metrics in literature. For more information, check the presentation below in Asilomar. More details can further be found in the paper (available on ArXiv), or by contacting the authors.
Video Presentation
DeepMIMO Dataset Scenario of ASU Campus
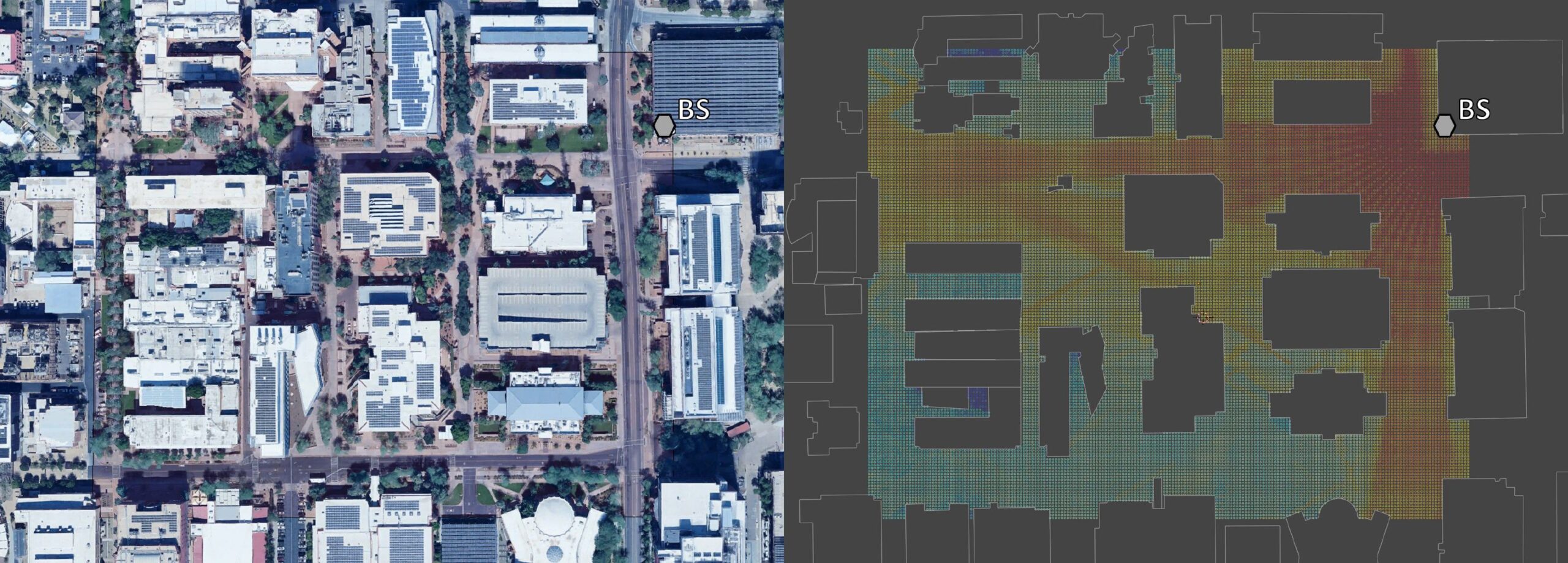
Fig. 3. Real (left) and rendered (right) top view the ASU campus DeepMIMO dataset. The base station is showed in both figures. It should be noted that buildings and other scenario assets are 3D, and their heights matter significantly for roof diffractions. The mesh represented in the synthetic counterpart represents the received power when applying a standard DFT codebook at the base station.
This dataset was generated with DeepMIMO and can be found in DeepMIMO ASU Campus 1.
DeepMIMO is a framework for generating large-scale MIMO datasets based on 3D Ray-tracing.
A DeepMIMO dataset is fully defined by (i) the ray-tracing scenario and (ii) the set of generation parameters.
DeepMIMO enables a wide range of machine/deep learning communication and sensing applications.
Reproduce the Results
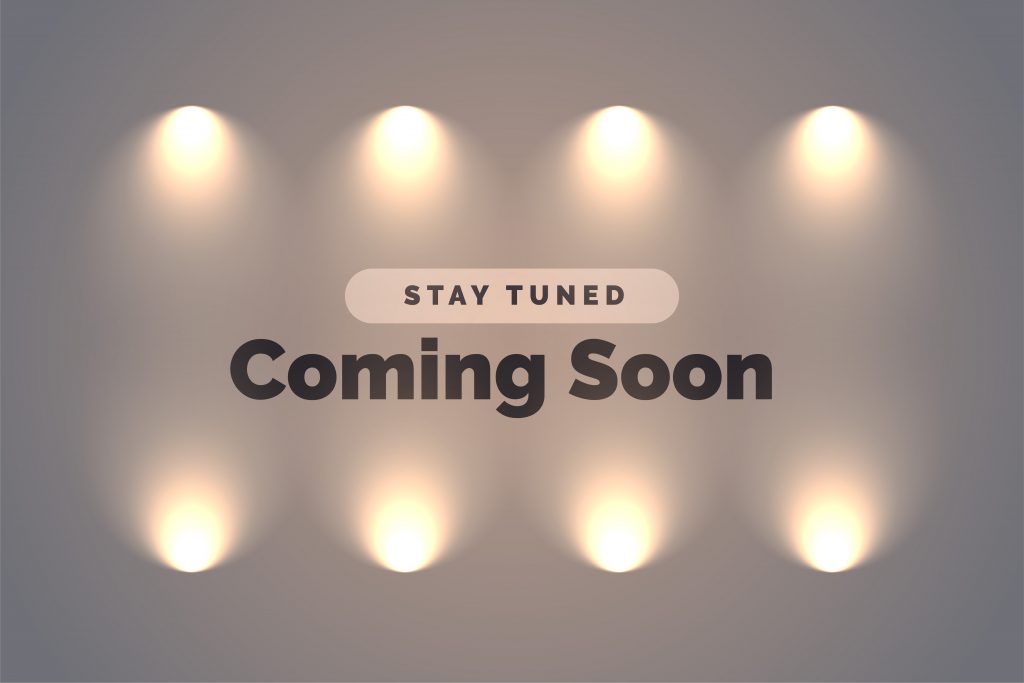
Citation
J. Morais, S. Alikhani, A. Malhotra, S. Hamidi-Rad, and A. Alkhateeb, “A Dataset Similarity Evaluation Framework for Wireless Communications and Sensing”, (To appear in Asilomar Conference on Signals, Systems, and Computers, Pacific Grove, CA, USA, 2024)
@INPROCEEDINGS{Joao2024DatasetSimilarity,
title={A Dataset Similarity Evaluation Framework for Wireless Communications and Sensing},
author={Morais, Joao and Alikhani, Sadjad and Malhotra, Akshay and Hamidi-Rad, Shahab and Alkhateeb, Ahmed},
journal={58th Asilomar Conference in Signals, Systems, and Computers},
year={2024}
}