Digital Twin Based Beam Prediction:
Can we Train in the Digital World and Deploy in Reality?
Published at IEEE ICC Workshops 2023
Shuaifeng Jiang and Ahmed Alkhateeb
Wireless Intelligence Lab, Arizona State University, USA
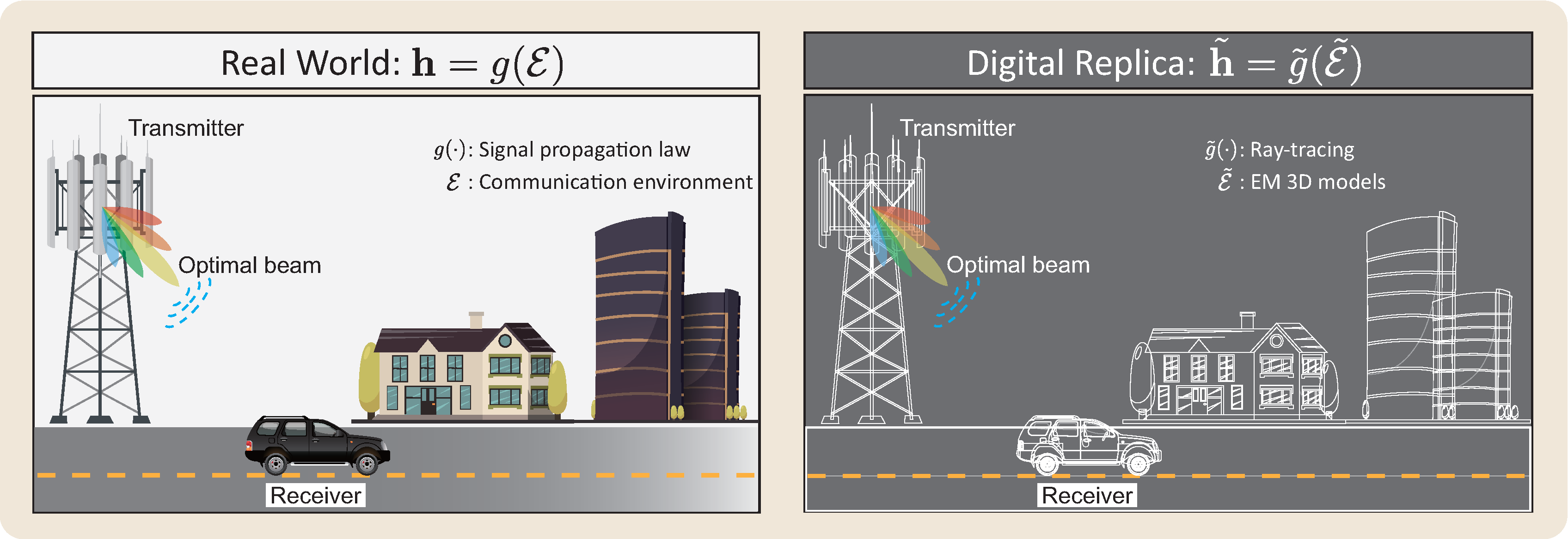
Fig. 1. This figure shows a real-world communication system and its digital replica. In the real world, the channel between the transmitter and the receiver is
determined by the communication environment and the signal propagation law. The digital replica employs the 3D model to approximate the communication environment and the ray tracing to model the signal propagation.
Abstract
Realizing the potential gains of large-scale MIMO systems requires the accurate estimation of their channels or
the fine adjustment of their narrow beams. This, however, is typically associated with high channel acquisition/beam sweeping overhead that scales with the number of antennas. Machine and deep learning represent promising approaches to overcome these challenges thanks to their powerful ability to learn from prior observations and side information. Training machine and deep learning models, however, requires large-scale datasets that are expensive to collect in deployed systems. To address this challenge, we propose a novel direction that utilizes digital replicas of the physical world to reduce or even eliminate the MIMO channel acquisition overhead. In the proposed digital twin aided communication, 3D models that approximate the real-world communication environment are constructed and accurate ray-tracing is utilized to simulate the site-specific channels. These channels can then be used to aid various communication tasks. Further, we propose to use machine learning to approximate the digital replicas and reduce
the ray tracing computational cost. To evaluate the proposed digital twin based approach, we conduct a case study focusing on the position-aided beam prediction task. The results show that a learning model trained solely with the data generated by the digital replica can achieve relatively good performance on the real-world data. Moreover, a small number of real-world data points can quickly achieve near-optimal performance, overcoming the modeling mismatches between the physical and digital worlds and significantly reducing the data acquisition overhead.
Simulation Results
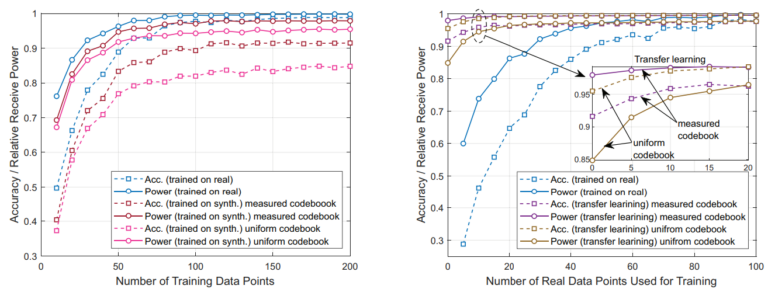
Fig. 2. Left shows the beamforming performance obtained by training the NN on the real-world (real) or digital twin (synthetic) data and testing on the real-world data. Right shows the performance by applying transfer learning to the NN model using the measured and the uniform beamforming codebooks.
Citation
Shuaifeng Jiang, and Ahmed Alkhateeb, ‘Digital Twin Based Beam Prediction: Can we Train in the Digital World and Deploy in Reality?’, IEEE ICC Workshops, 2023.
@inproceedings{jiang2023digital,
title={Digital twin based beam prediction: Can we train in the digital world and deploy in reality?},
author={Jiang, Shuaifeng and Alkhateeb, Ahmed},
booktitle={2023 IEEE International Conference on Communications Workshops (ICC Workshops)},
pages={36–41},
year={2023},
organization={IEEE}
}